Reproducible analysis
Last updated on 2025-07-01 | Edit this page
Estimated time: 60 minutes
Overview
Questions
- How do I make my research project reproducible?
- How do I include packages as dependencies of my project?
- What features are related to reproducible research?
Objectives
- Add dependencies of a project using the
DESCRIPTION
file. - Create an isolated and specific reproducible environment with renv
- Identify your project features related to reproducible software.
How do I make my analysis reproducible?
The reproducible environment
Any analysis with R needs packages. These packages on which your
project relies are called dependencies.
To make an analysis reproducible, we need to register these packages
(and their versions) somewhere as your project’s dependencies. That
place is the DESCRIPTION
file.
In the DESCRIPTION
file, dependencies are registered at the end of the file with the
package names only and usually with a minimum version
(dplyr (>= 1.0.0)
). We can add dependencies using
functions (rcompendium::add_dependencies()
), and also use
this file to automate version recovery
(devtools::install_deps()
). However,
DESCRIPTION
files are most useful for R packages.
For non-package
projects we can use renv. It registers specific
dependencies by implementing project-specific environments, which means
that renv registers even the SHA/hash
from GitHub packages, feature that the DESCRIPTION
file can
not do. Also, renv isolates your project packages from
your computer packages. Lastly, renv can detect new
dependencies automatically, apart from adding them with functions
(renv::snapshot()
), and it can also automate the recovery
of the whole project (renv::restore()
).
Callout
The renv package:
- Isolates the dependencies of your project from your computer.
- Registers the specific version of packages from CRAN or GitHub.
- Provides an automated package management solution to restore an external project.
The analysis workflow
Complementary to the dependencies, your analysis workflow must follow some good practices in scientific computing.
First, for Data management, we need to save input
data as originally created and, preferably, configure it as a read-only
file. In your project, you can differentiate raw-data
from
derived-data
Second, for Project organization, we need to store
analysis and generated files in specific and isolated folders. In your
project, you can differentiate analyses
files (like
.R
scripts and .Rmd
files) from
figures
and other outputs
.
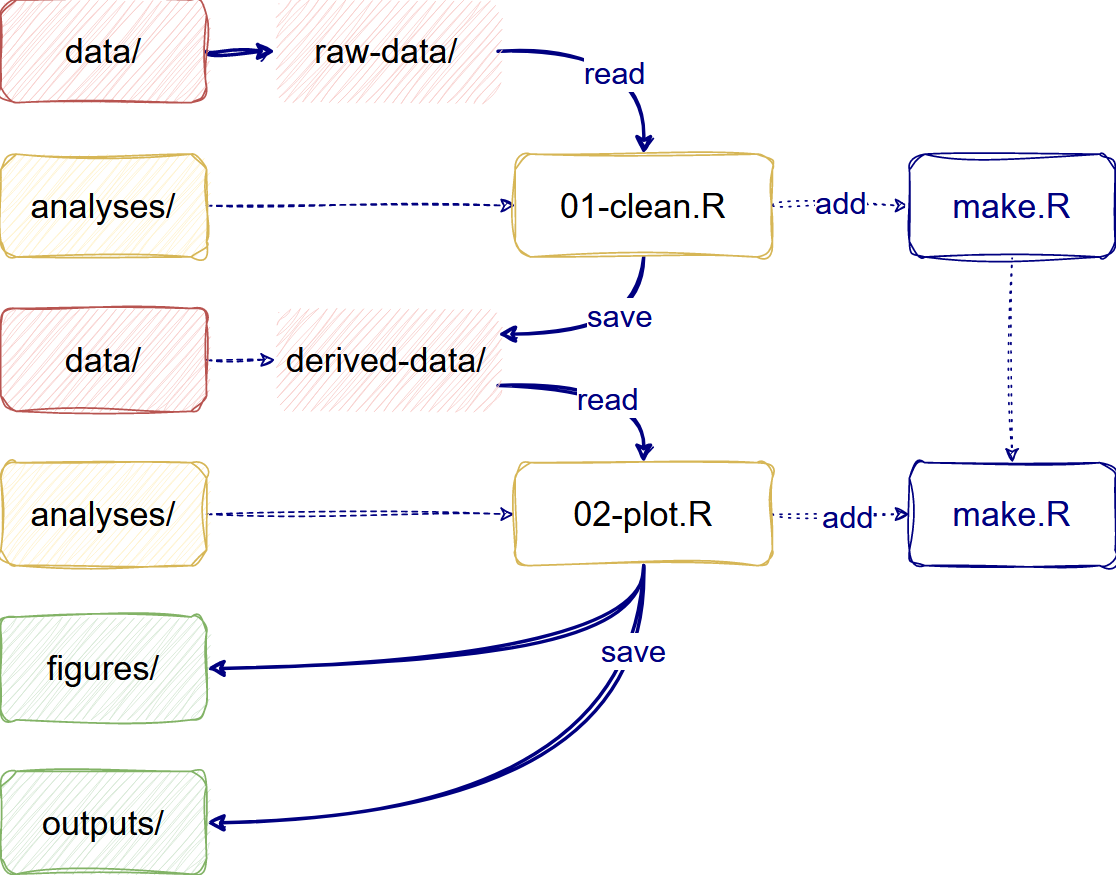
Automate your analysis
The make.R
file helps automate your analysis project.
This file includes a script line to automatically restore your
dependencies (renv::restore()
) and run all the analysis
scripts in your preferred order. The make.R
file is the
only .R
file stored in the project’s root given by the
rcompendium template. You can use the make.R
file as the only script to run and regenerate all your project
outputs.
Callout
The make.R
file is inspired but not equivalent to GNU
Make
file.
GNU
Make
files can identify out-of-date files and
re-execute any downstream code that needs to be updated, usually used
for bash
scripts.
To use this functionality for your R
project, you can
use the {targets}
package.
Let’s code
We need to play under the rules of the rcompendium template.
The reproducible environment
We will use renv instead of DESCRIPTION
files for this.
Usually, to initiate a
reproducible environment with renv, we need to run
renv::init()
.
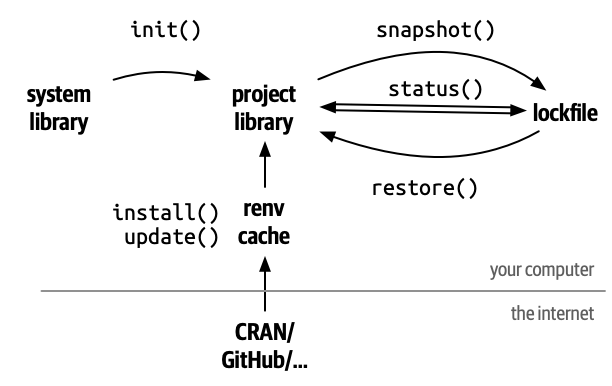
However, when working in a rcompendium template, your first step must be to run:
R
rcompendium::add_renv()
OUTPUT
This project contains a DESCRIPTION file.
Which files should renv use for dependency discovery in this project?
1: Use only the DESCRIPTION file. (explicit mode)
2: Use all files in this project. (implicit mode)
Write 2
and press ENTER to use renv
instead of DESCRIPTION
file.
Question
Why not to use renv in addition to
DESCRIPTION
?
We can use renv in addition to
DESCRIPTION
.
However, we opt to use renv instead of
DESCRIPTION
because the
rcompendium::add_dependencies(".")
function because it
assumes that all packages to add to DESCRIPTION
are from
CRAN. If you want to add GitHub packages, you need to
add them manually in a different section called
Remotes:
and write repository/package
. The
renv package solves this automatically.
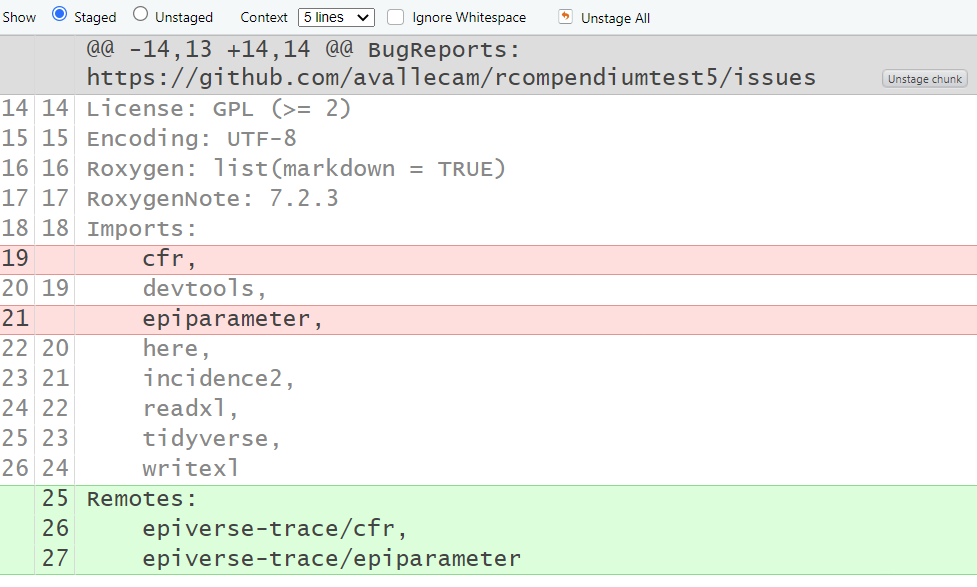
However, this still needs to be assessed with different scenarios to confirm this as the final best decision.
If you decide to use renv in addition to
DESCRIPTION
run:
R
rcompendium::add_dependencies(".")
Note that this function requires one argument specification
"."
, which means that your working
directory must be at the root of the R project.
The output below details which packages were included in the description file
✔ Scanning 'Imports' dependencies
(*) Found 2 package(s)
(*) Adding the following line in 'DESCRIPTION': `Imports: devtools, here`
If you get an error message like:
ERROR
Error in renv_snapshot_validate_report(valid, prompt, force) :
aborting snapshot due to pre-flight validation failure
Run again the rcompendium::add_renv()
function. You may
get the following message:
OUTPUT
This project already has a private library. What would you like to do?
1: Activate the project and use the existing library.
2: Re-initialize the project with a new library.
3: Abort project initialization.
Write option 1
and press ENTER.
This step creates a renv/
folder and modifies the
content of the make.R
in line 15
, replacing
the default devtools::install_deps()
by
renv::restore
.
Second, to get the status of the project run:
R
renv::status()
OUTPUT
This project does not contain a lockfile.
Use renv::snapshot() to create a lockfile.
Callout
Always follow the suggestions of the renv::status()
output. You can also get a message from it each time you reopen your
project.
Third, to create the lockfile
run:
R
renv::snapshot()
This step creates a renv.lock
file detailing the
following:
- R version on top and
- specific version details of all the packages in the project’s dependency tree (including SHA/hash for GitHub packages).
{
"R": {
"Version": "4.2.2",
"Repositories": [
{
"Name": "CRAN",
"URL": "https://packagemanager.posit.co/cran/latest"
}
]
},
"Packages": {
"R6": {
"Package": "R6",
"Version": "2.5.1",
"Source": "Repository",
"Repository": "RSPM",
"Requirements": [
"R"
],
"Hash": "470851b6d5d0ac559e9d01bb352b4021"
},
...
Now, you have completed your reproducible environment configuration.
The analysis workflow
The workflow will follow these three paths:
- Read
raw-data/
toclean.R
it and save it toderived-data/
. - Read
derived-data/
to make aplot.R
and save it tofigures/
. - Read
derived-data/
to make atable.R
and save it tooutputs/
.
First, download the sample data set.
Since this is raw data, save it in the data/raw-data/
folder.
Second, create the analysis script to clean this raw data set. Name
it 01-clean.R
. Save it in the analyses/
folder. Copy and paste these lines of code:
R
# Load packages
library(readxl)
library(tidyverse)
# Read raw data
dat_path <- "https://github.com/reconhub/learn/raw/master/static/data/linelist_20140701.xlsx"
dat <- rio::import(file = dat_path) %>%
tibble::as_tibble()
# Clean raw data
dat_clean <- dat %>%
dplyr::select(case_id, date_of_onset, date_of_outcome, outcome) %>%
dplyr::mutate(dplyr::across(
.cols = c(date_of_onset, date_of_outcome),
.fns = as.Date
)) %>%
dplyr::mutate(
outcome = fct(outcome, level = c("Death", "Recover"), na = "NA")
)
# Write clean data
dat_clean %>%
readr::write_rds("outputs/linelist_clean.rds")
Notice that we are writing a new cleaned data set in a different
path: data/derived-data/
.
Callout
The default folder to save R scripts will be
R/
. This path is the place to write your Modular functions. Go to theanalyses/
folder to save your analysis script.Yes, it is named
analyses/
not “analysis”.
Rstudio will invite you to install new packages. Press Install.
Always run renv::status()
after installing new
packages:
R
renv::status()
OUTPUT
The following package(s) are in an inconsistent state:
package installed recorded used
backports y n y
bit y n y
In this case, we need to follow the instructions in the section of Missing
packages from the ?renv::status()
documentation.
R
renv::install()
OUTPUT
- There are no packages to install.
- Automatic snapshot has updated '~/0projects/projectname/renv.lock'.
Third, create an analysis script to create an incidence plot for
this cleaned data set. Name it 02-plot.R
. Save it in the
analyses/
folder. Copy and paste these lines of code:
R
# Load packages
library(tidyverse)
library(incidence2)
# Read data
ebola_dat <- readr::read_rds("outputs/linelist_clean.rds")
# Create incidence2 object
ebola_onset <-
incidence2::incidence(
x = ebola_dat,
date_index = c("date_of_onset"),
interval = "epiweek"
)
# Read incidence2 object
ebola_onset
# Plot incidence data
plot(ebola_onset)
# Write ggplot as figure
ggsave("figures/02-plot_incidence.png", height = 3, width = 5)
Notice that we are writing the new plot in a different path:
figures/
.
Challenge
- Explore the
i2extras::fit_curve()
to fit a model to the incidence curve. - Save the output table in the corresponding folder.
- You can reuse the
incidence2
object as input in the same file. - Remember to update the renv status if you need to install and use a new package for this task
Automate your analysis
The easiest step to forget!
Lastly, list all .R
scripts and .Rmd
in a
sequential order in the make.R
file after
line 32
:
R
## Run Project ----
# List all R scripts in a sequential order and using the following form:
# source(here::here("analyses", "script_X.R"))
source(here::here("analyses", "01-clean.R"))
source(here::here("analyses", "02-plot.R"))
Checklist
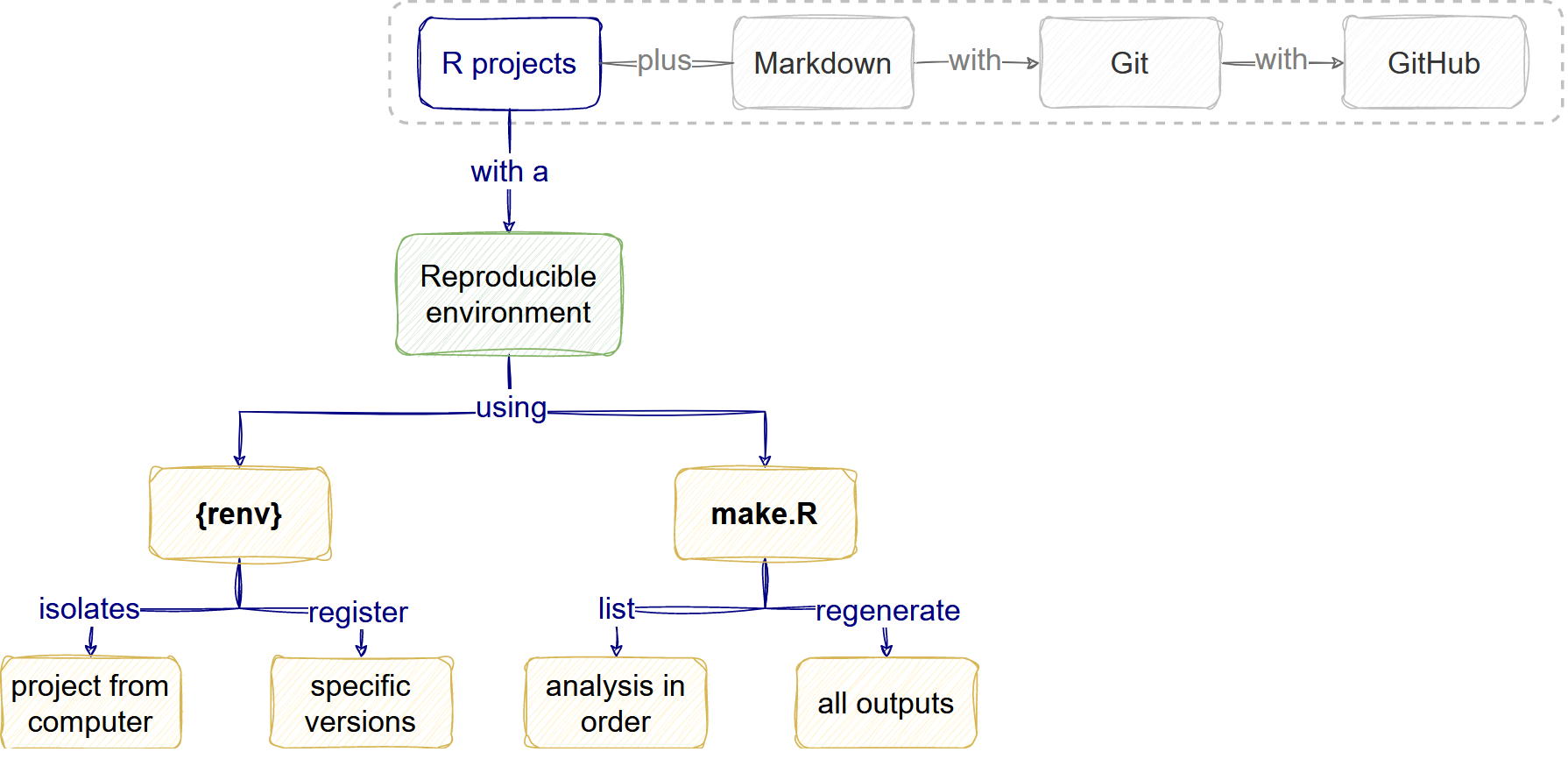
Reproducible research features
We defined Reproducible research as a practice that wants to ensure that anyone with access to data inputs and software can feasibly generate the outputs to check or build on them.
A key feature of this practice is the combination of
renv with the
make.R
file. With this file, and any other
more sophisticated alternatives like GNU Make
or
targets, we are sure that we:
- Can feasibly regenerate the outputs.
- Can inform about the reliability of the project.
- Have an isolated time-proof capsule of dependencies.
Key Points
A dependency is a package that your project needs to run.
Use the
DESCRIPTION
file to register your project dependencies.Use renv to isolate and create package-specific reproducible environments for your dependencies.
Use the folder template to differentiate your
raw-data/
andderived-data/
.Save analysis and generated files in isolated folders like
analyses/
,figures/
, andoutputs/
.Use the
make.R
to list your analysis scripts and facilitate the regeneration of all your outputs.Reproducible environments and Make files are features related to Reproducible research.