Access epidemiological delay distributions
Figure 1
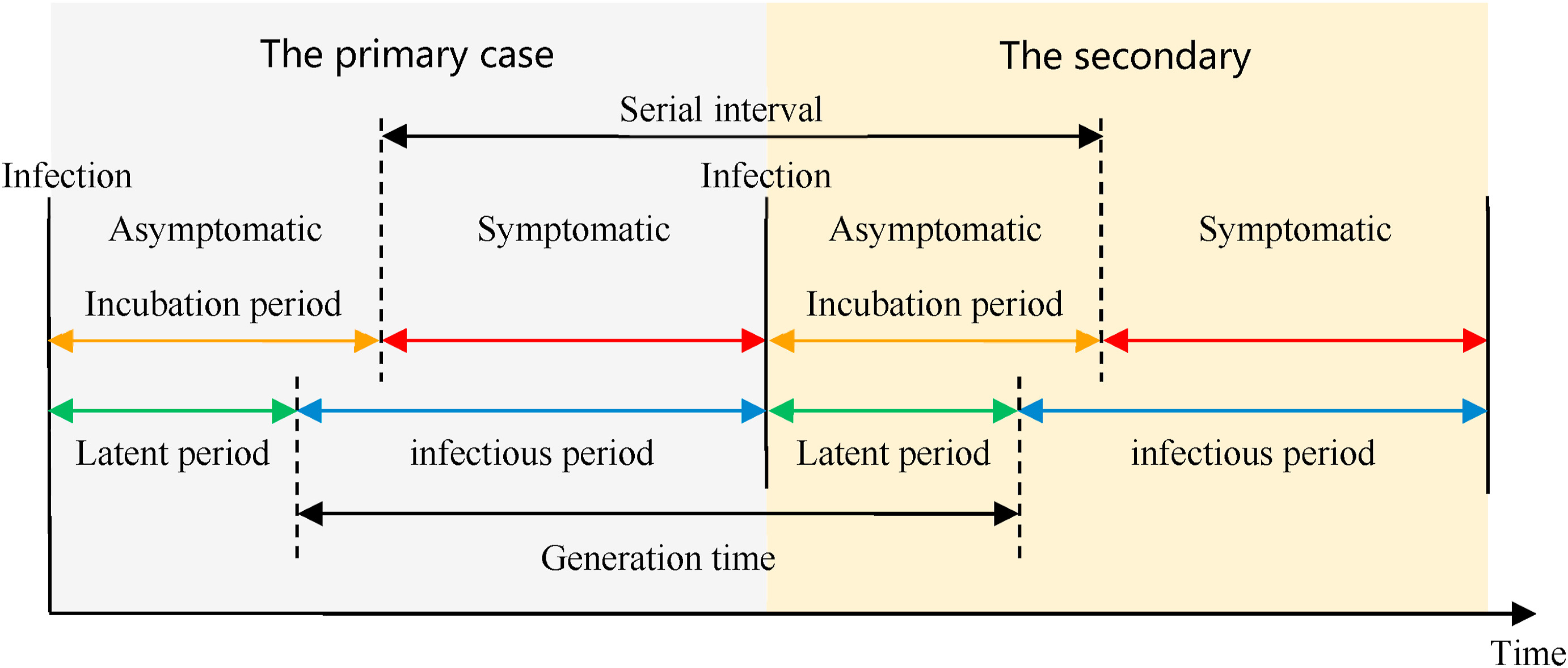
Definition of key time periods. From Xiang
et al, 2021
Figure 2
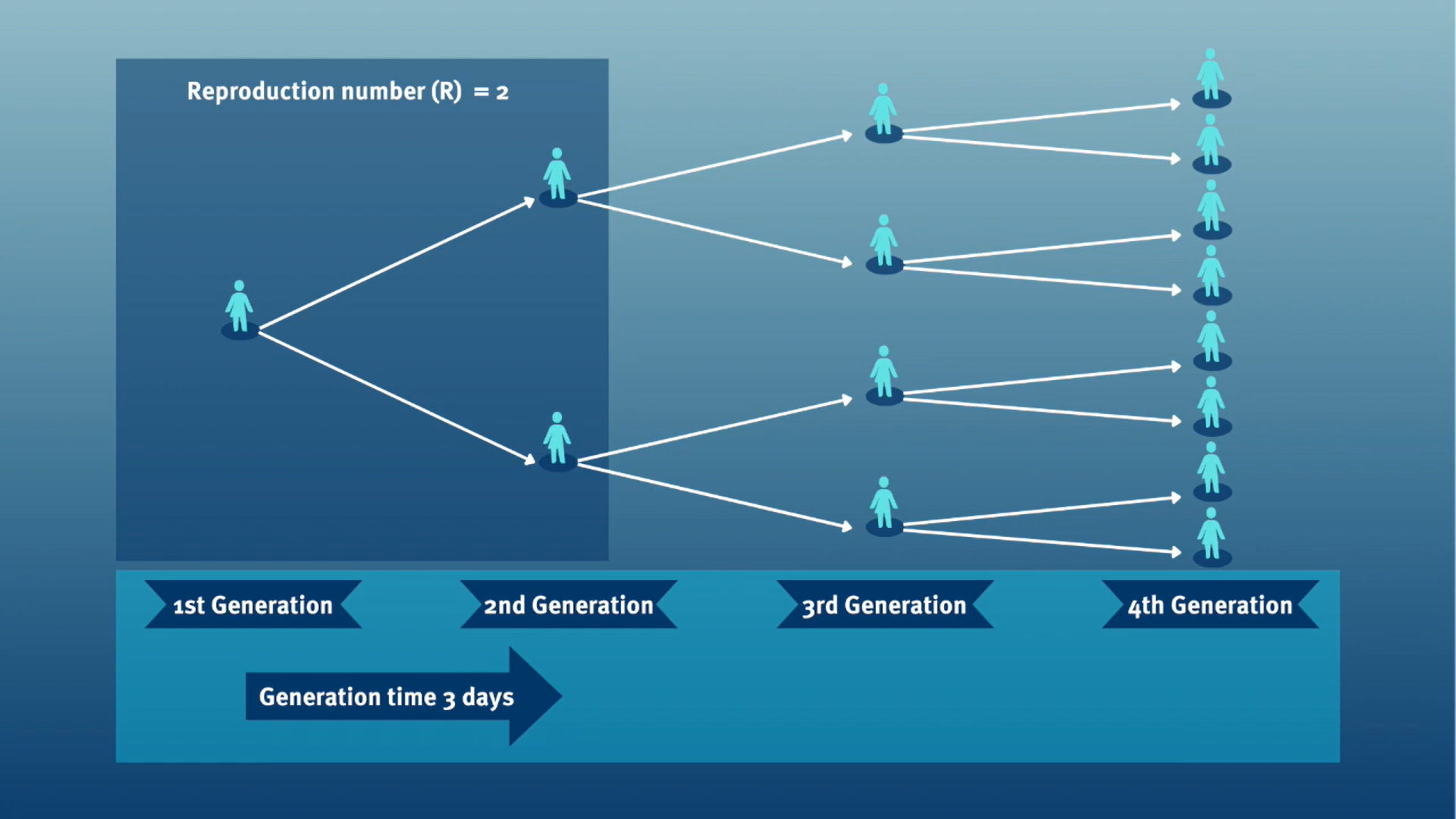
Video from the MRC Centre for Global Infectious
Disease Analysis, Ep 76. Science In Context - Epi Parameter Review Group
with Dr Anne Cori (27-07-2023) at https://youtu.be/VvpYHhFDIjI?si=XiUyjmSV1gKNdrrL
Figure 3
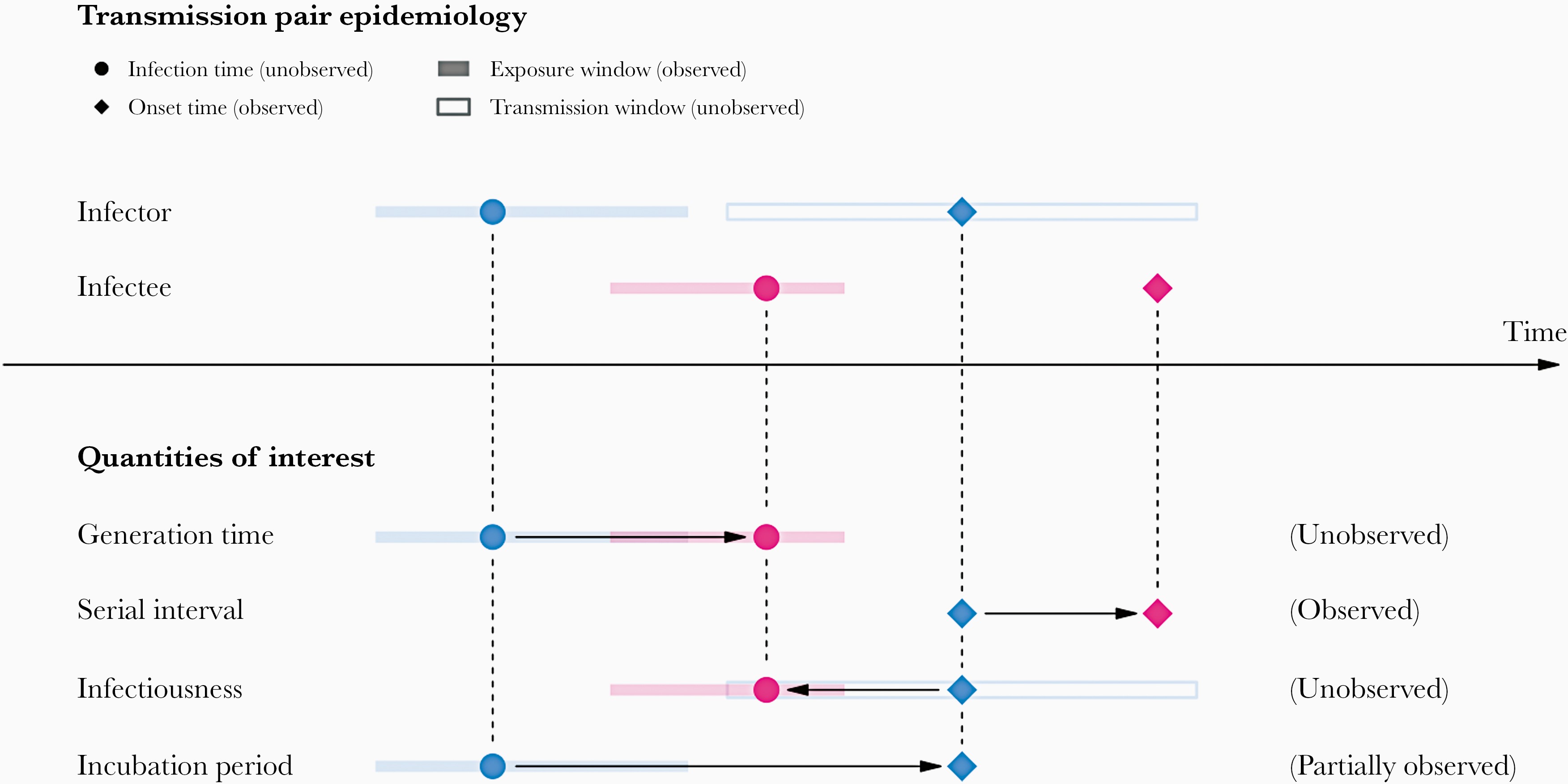
A schematic of the relationship of different
time periods of transmission between an infector and an infectee in a
transmission pair. Exposure window is defined as the time interval
having viral exposure, and transmission window is defined as the time
interval for onward transmission with respect to the infection time (Chung
Lau et al., 2021).
Figure 4
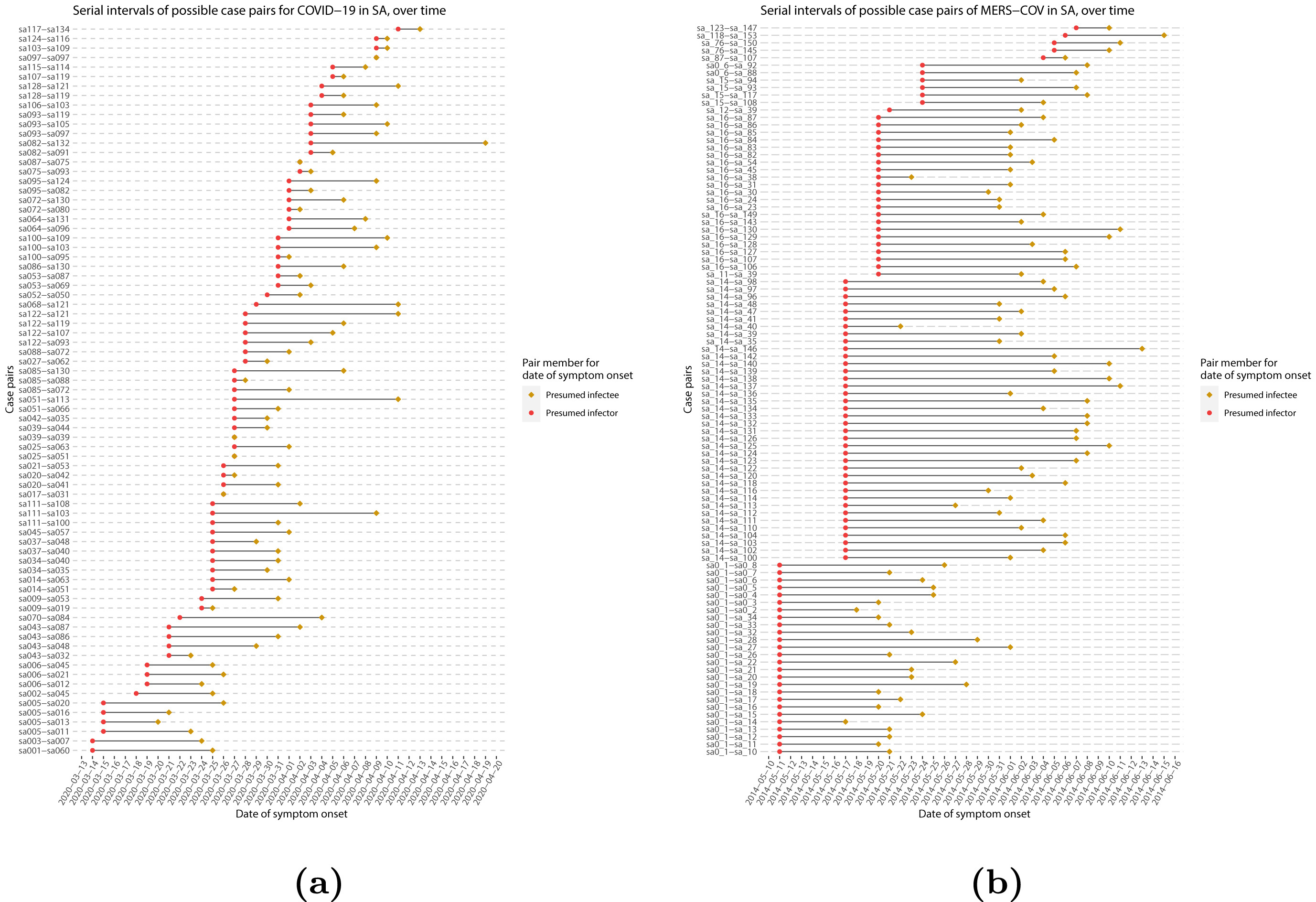
Serial intervals of possible case pairs in (a)
COVID-19 and (b) MERS-CoV. Pairs represent a presumed infector and their
presumed infectee plotted by date of symptom onset (Althobaity
et al., 2022).
Figure 5
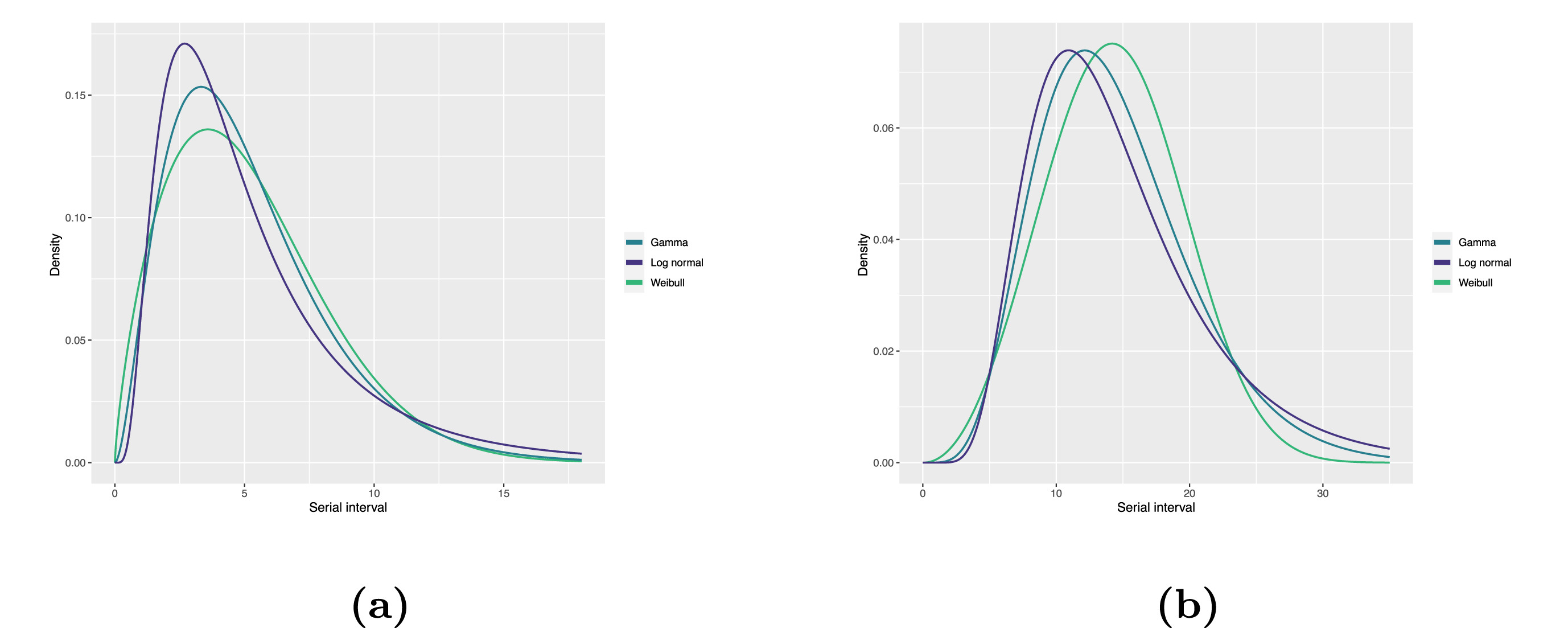
Fitted serial interval distribution for (a)
COVID-19 and (b) MERS-CoV based on reported transmission pairs in Saudi
Arabia. We fitted three commonly used distributions, Log normal, Gamma,
and Weibull distributions, respectively (Althobaity
et al., 2022).
Figure 6
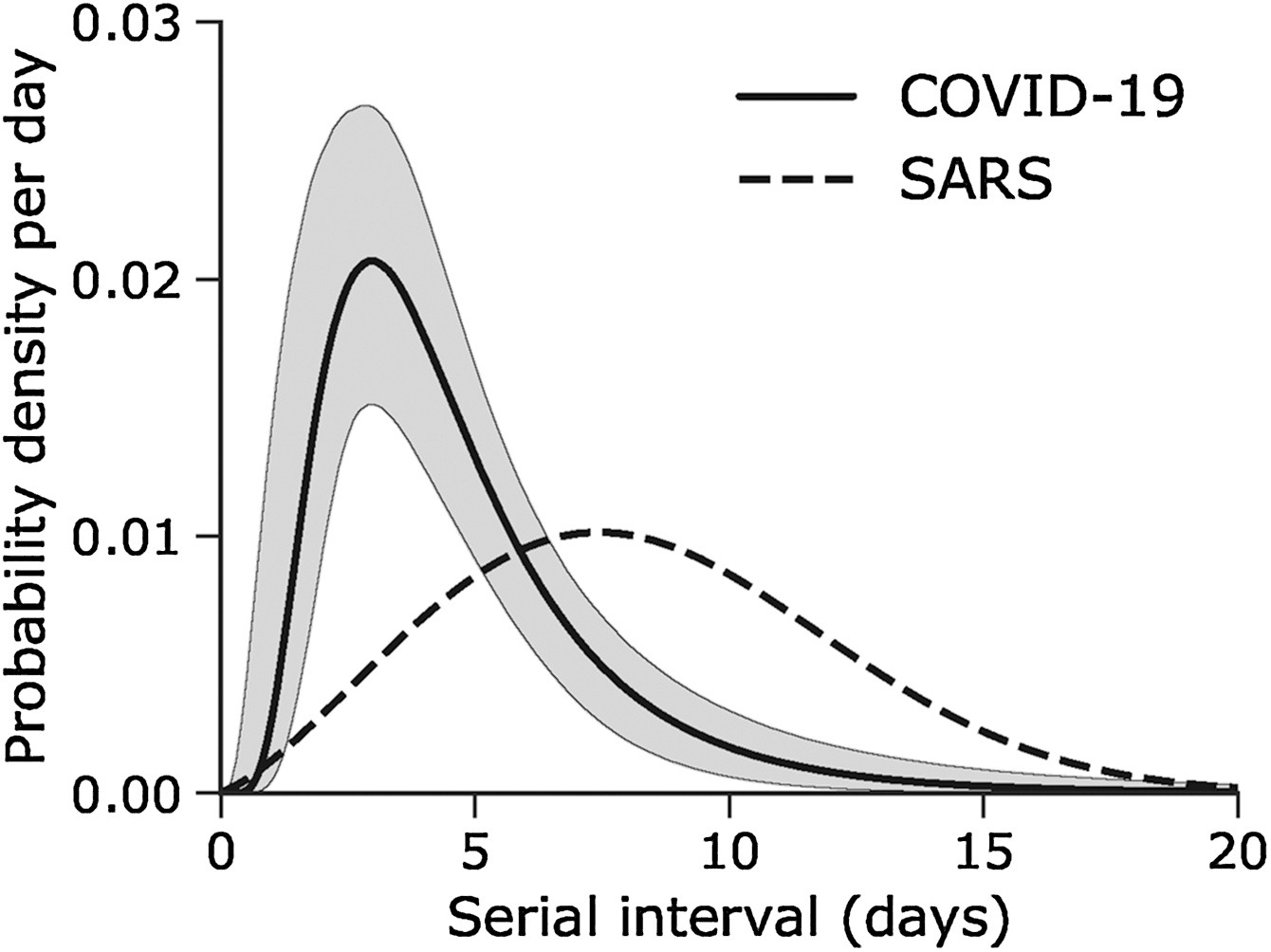
Serial interval of novel coronavirus (COVID-19)
infections overlaid with a published distribution of SARS. (Nishiura
et al., 2020)
Figure 7
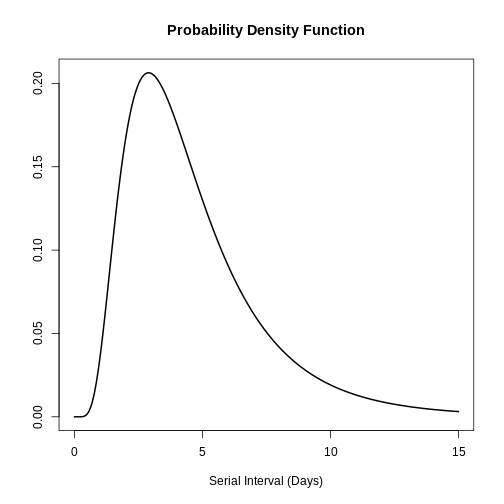
Quantifying transmission
Figure 1
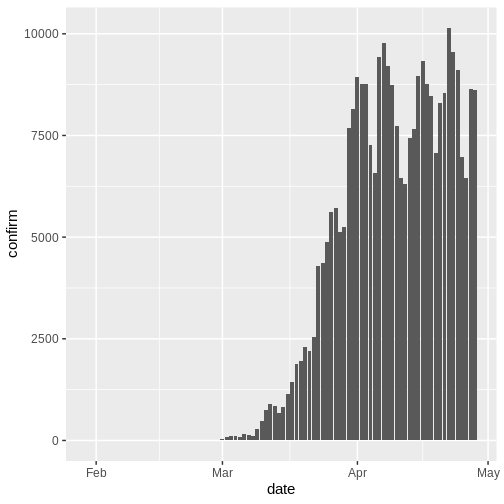
Figure 2
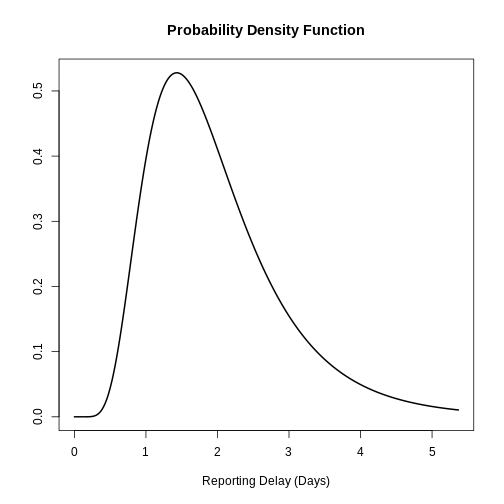
Figure 3
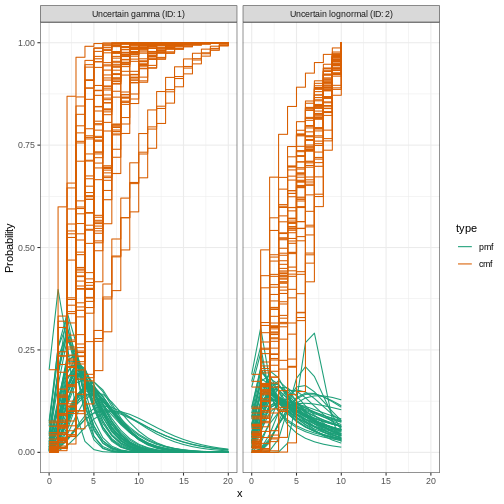
Figure 4
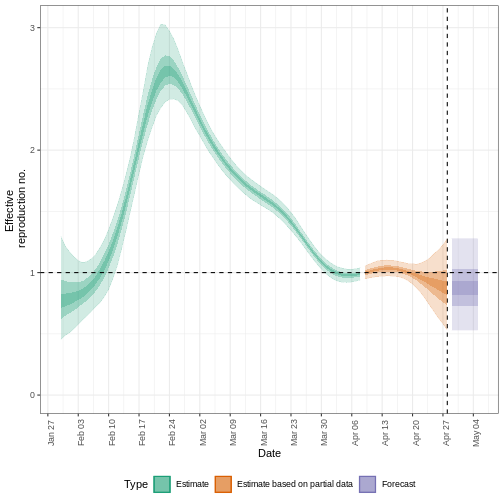
Figure 5
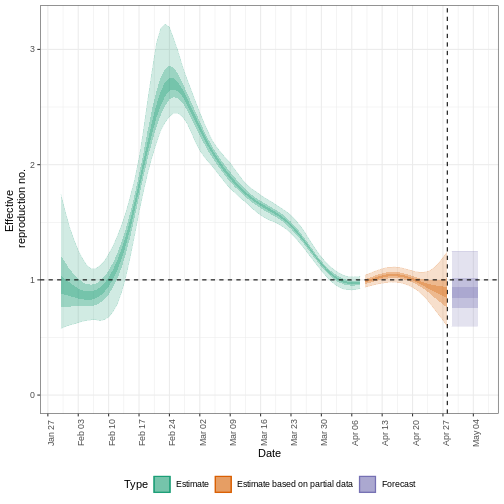
Figure 6
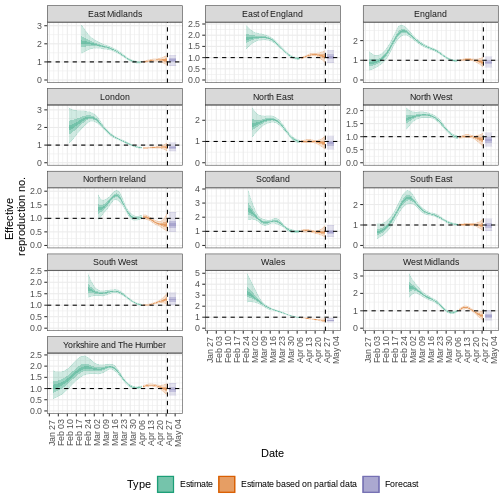
Use delay distributions in analysis
Figure 1
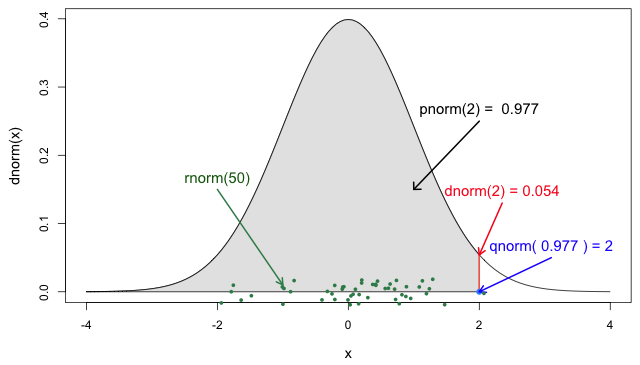
The four probability functions for the normal
distribution (Jack
Weiss, 2012)
Figure 2
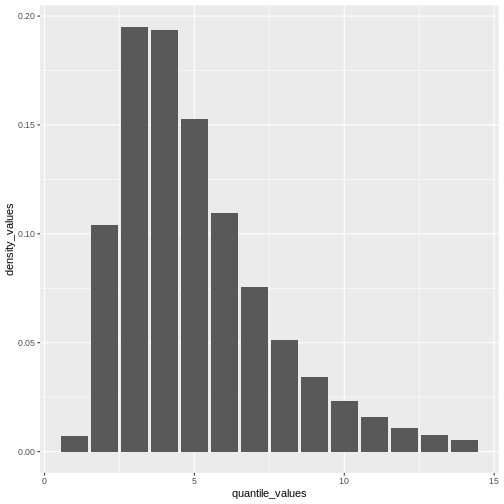
Figure 3
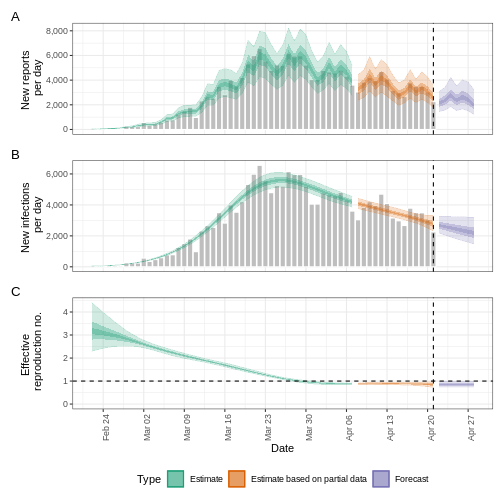
Figure 4
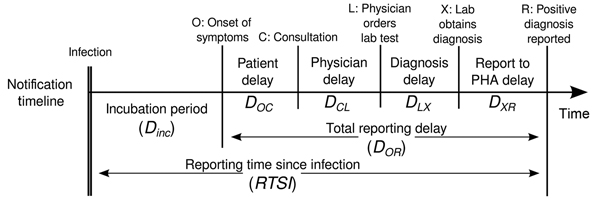
Timeline for chain of disease reporting,
the Netherlands. Lab, laboratory; PHA, public health authority.
From Marinović
et al., 2015
Figure 5
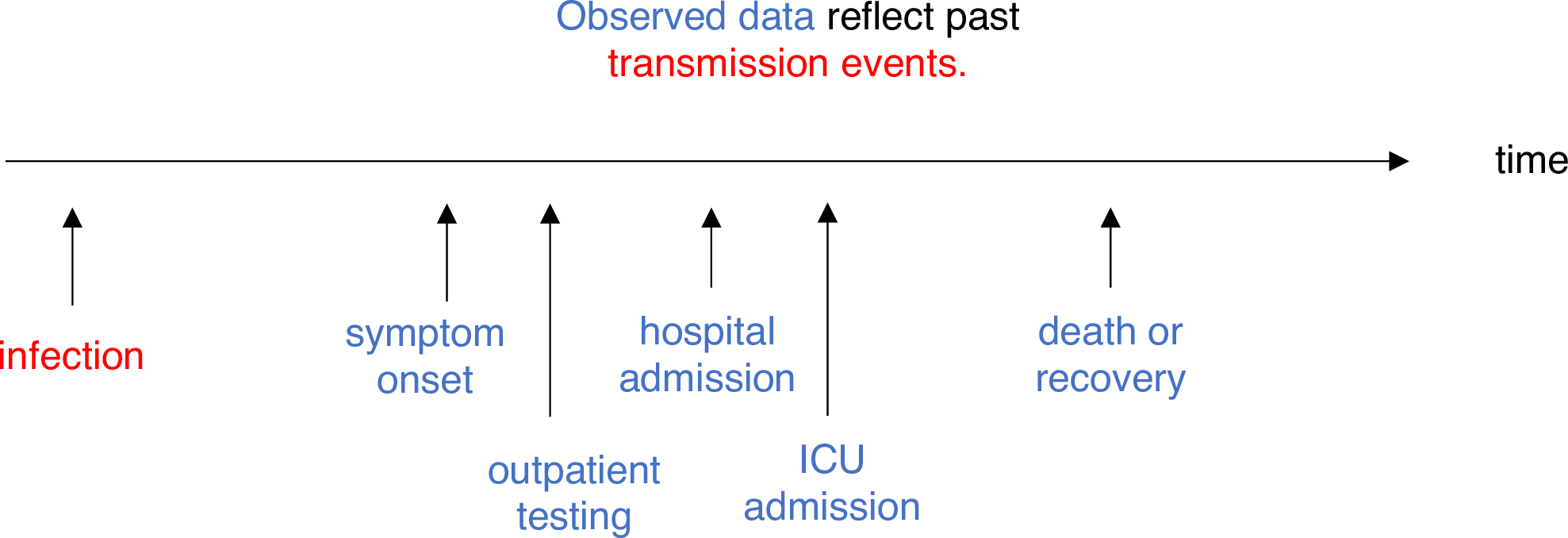
\(R_{t}\) is a measure of transmission at
time \(t\). Observations after
time \(t\) must be adjusted. ICU,
intensive care unit. From Gostic
et al., 2020
Figure 6
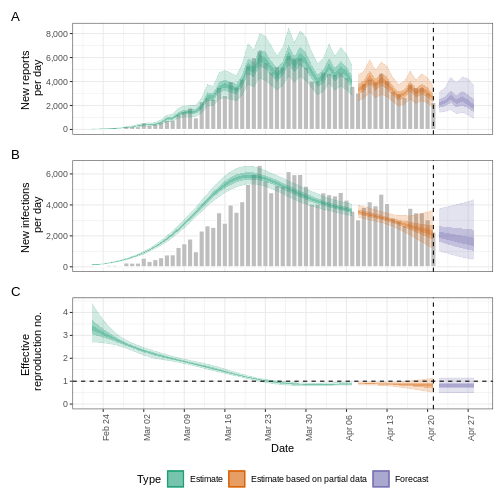
Figure 7
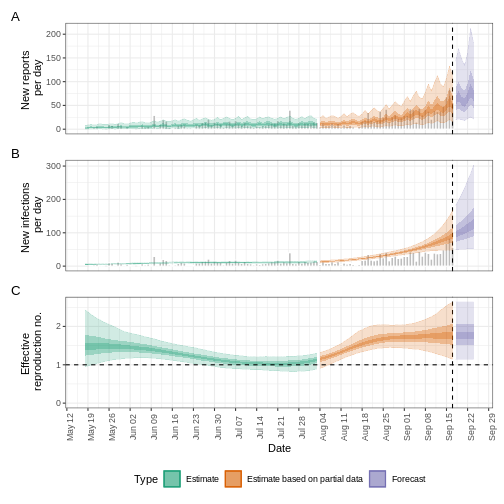
Figure 8
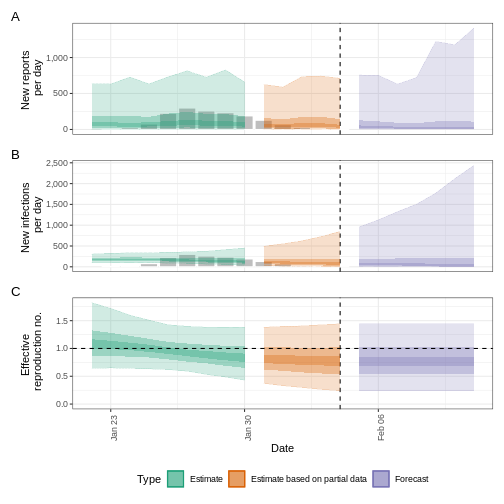
Create a short-term forecast
Figure 1
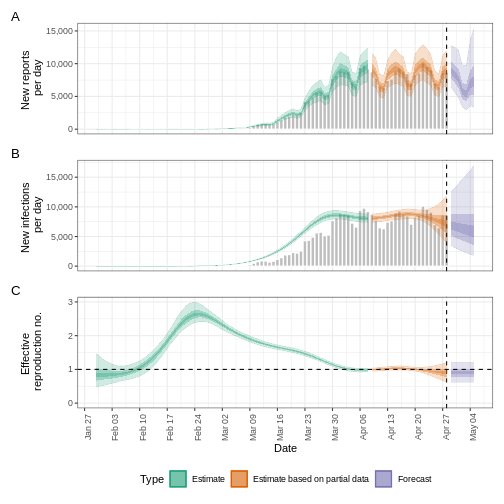
Figure 2
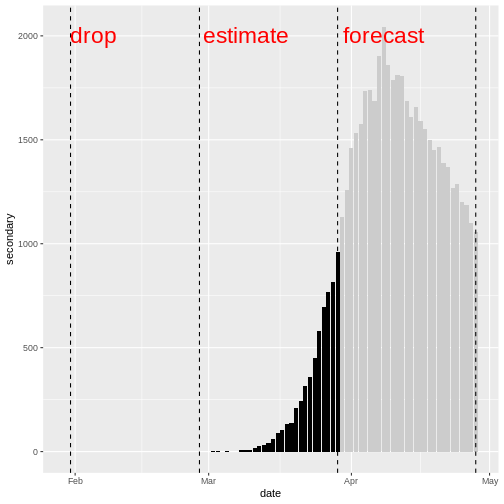
Figure 3
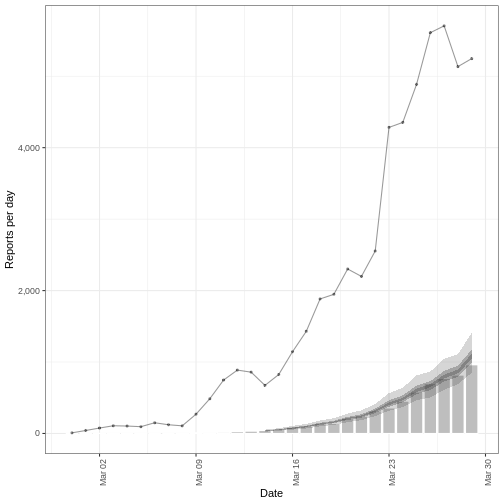
Figure 4
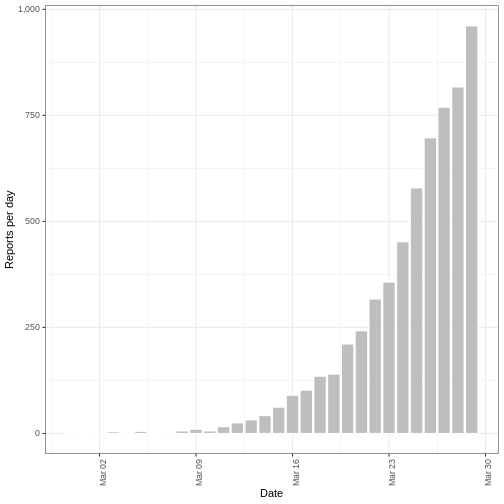
Estimation of outbreak severity
Figure 1
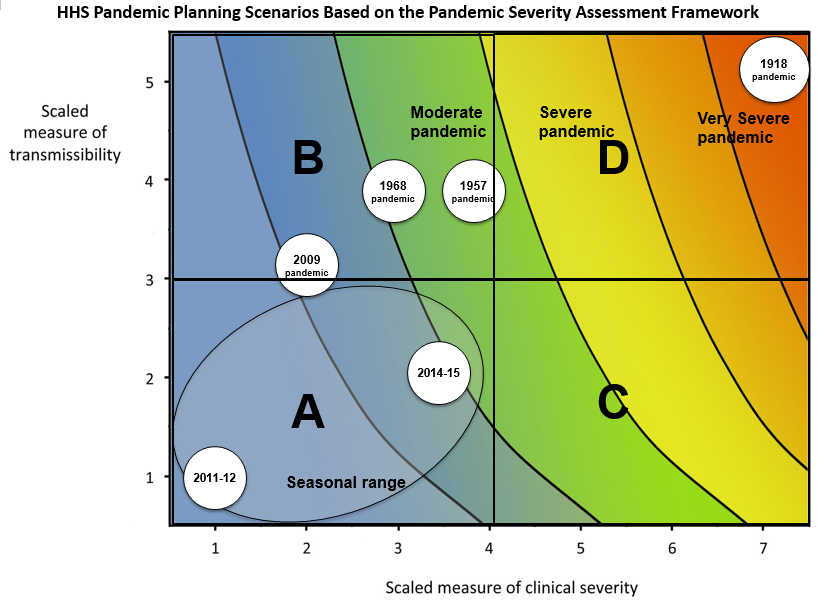
HHS Pandemic Planning Scenarios based on the
Pandemic Severity Assessment Framework. This uses a combined measure of
clinical severity and transmissibility to characterise influenza
pandemic scenarios. HHS: United States Department of
Health and Human Services (CDC,
2016).
Figure 2
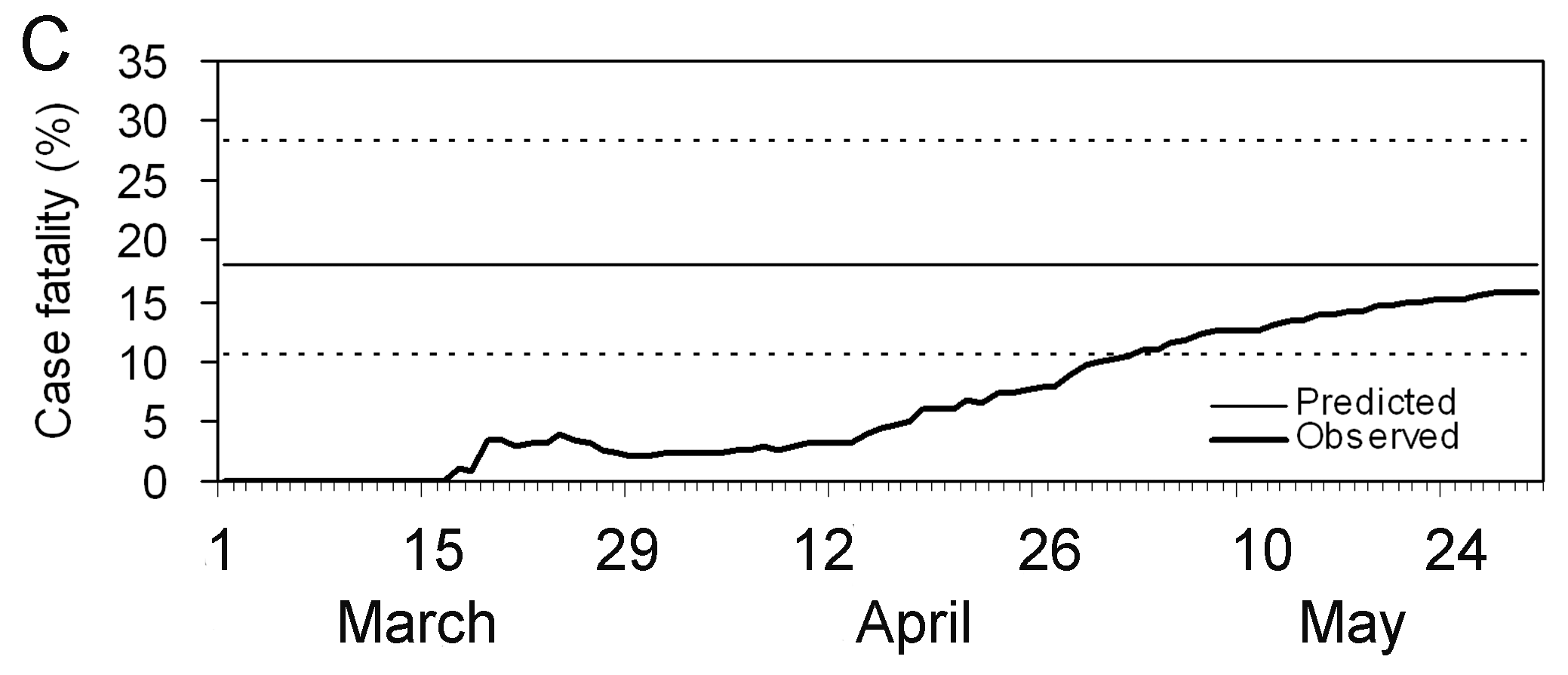
Observed biased confirmed case fatality risk
(CFR) estimates as a function of time (thick line) calculated as the
cumulative number of deaths over confirmed cases at time t. The estimate
at the end of an outbreak (~May 30) is the realised CFR by the end of
the epidemic. The horizontal continuous line and dotted lines show the
expected value and the 95% confidence intervals (\(95\%\) CI) of the predicted delay-adjusted
CFR estimate only by using the observed data until 27 Mar 2003 (Nishiura
et al., 2009)
Figure 3
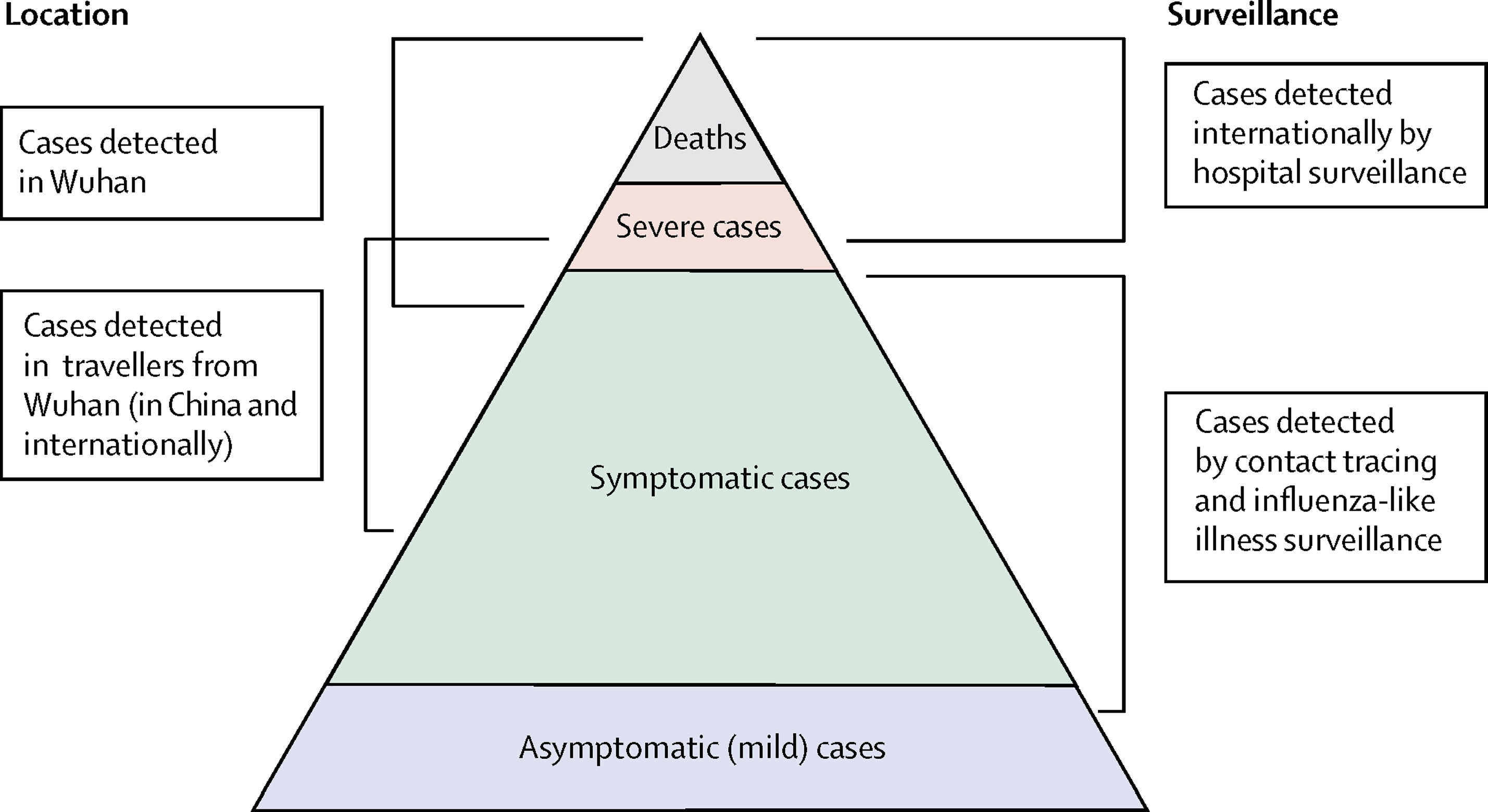
Spectrum of COVID-19 cases. The CFR aims to
estimate the proportion of Deaths among confirmed cases in an epidemic.
(Verity
et al., 2020)
Figure 4
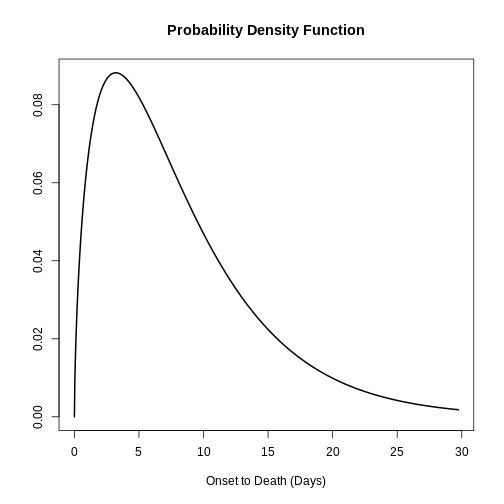
Figure 5
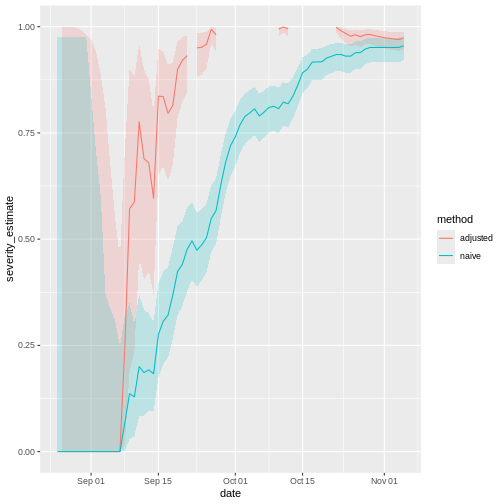
Figure 6
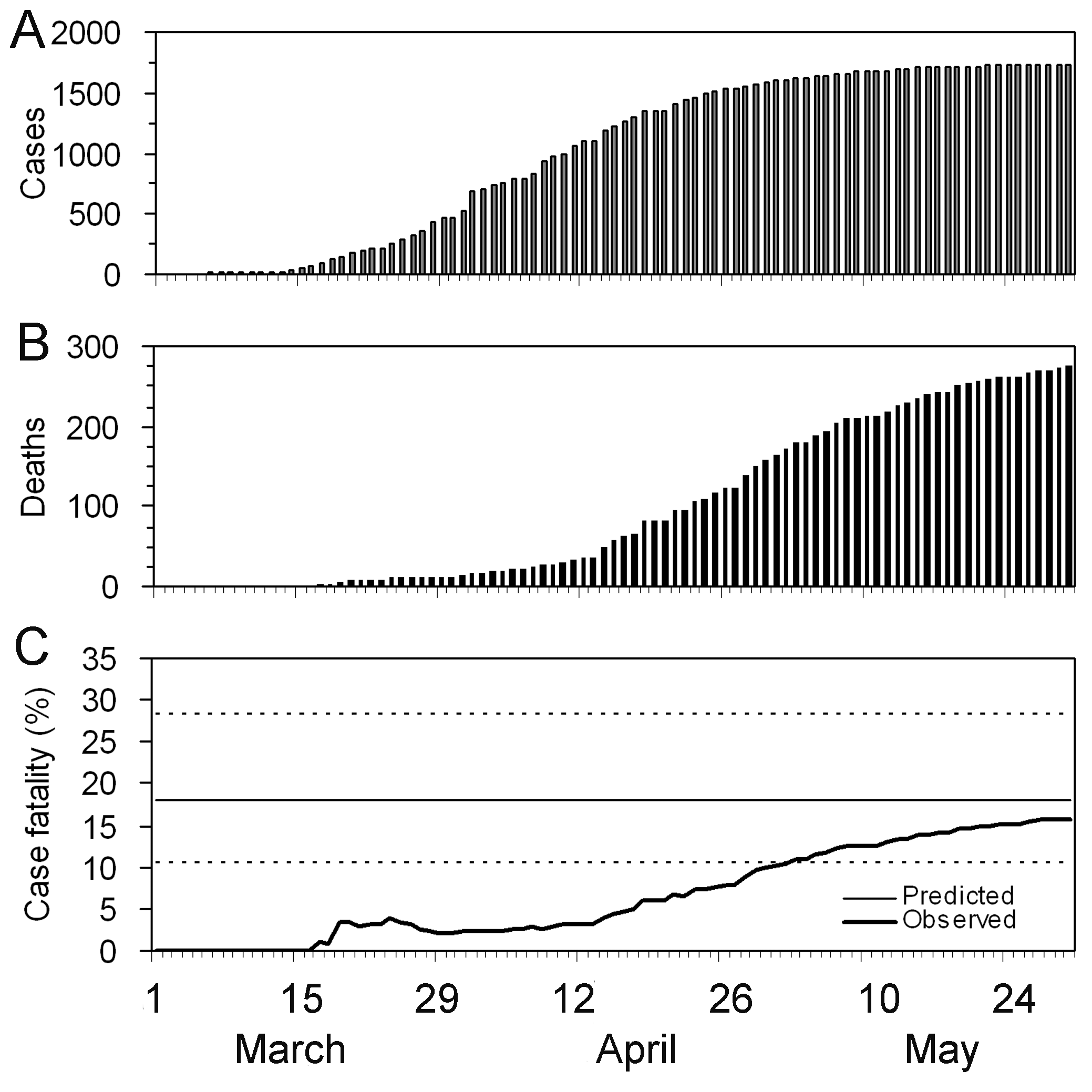
Observed (biased) confirmed case fatality risk
of severe acute respiratory syndrome (SARS) in Hong Kong, 2003. (Nishiura
et al., 2009)
Figure 7
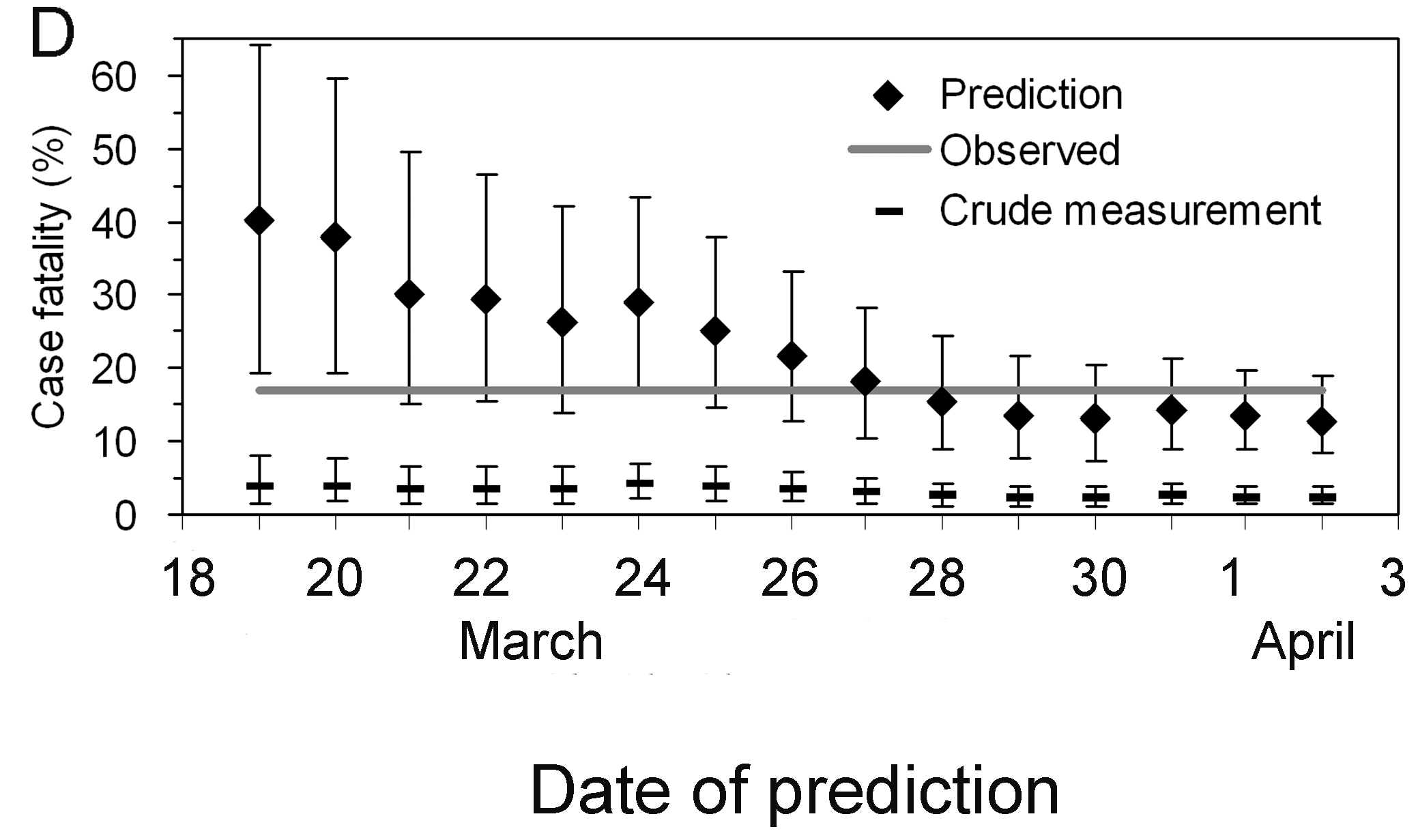
Early determination of the delay-adjusted
confirmed case fatality risk of severe acute respiratory syndrome (SARS)
in Hong Kong, 2003. (Nishiura
et al., 2009)
Figure 8
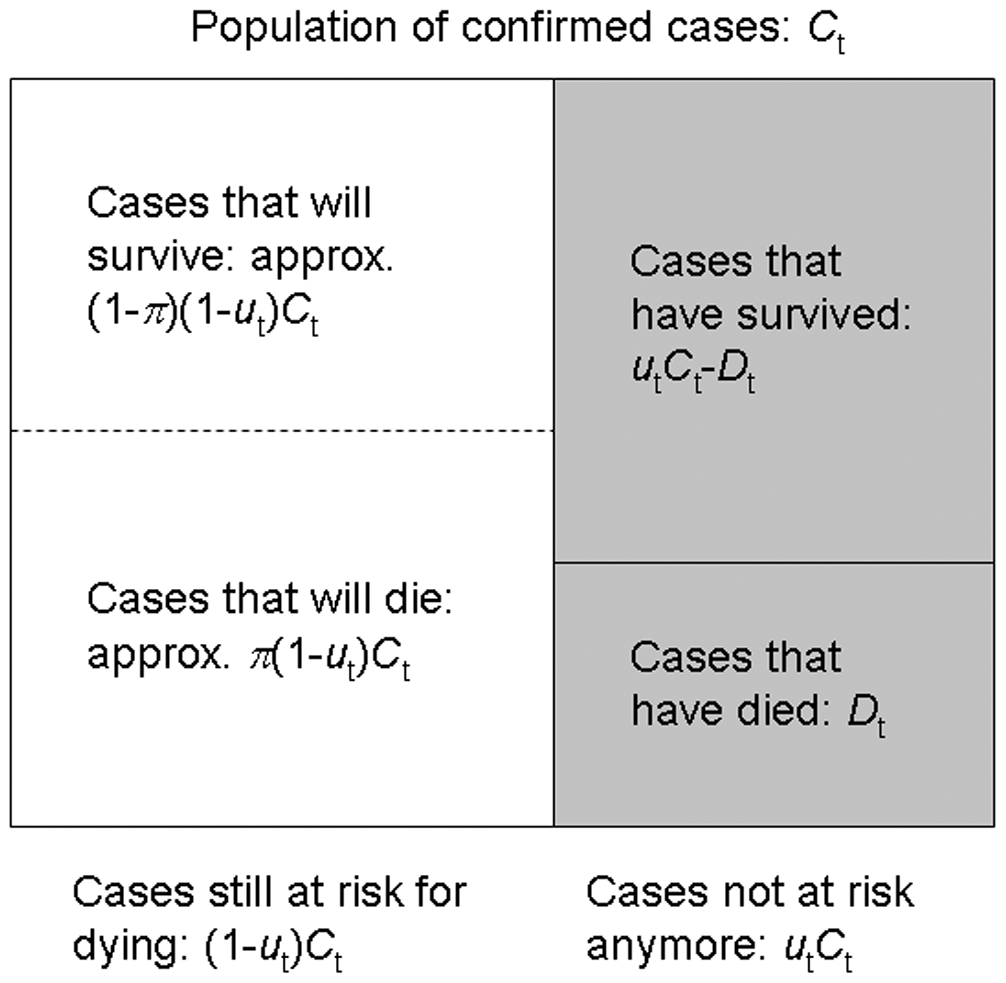
The population of confirmed cases and sampling
process for estimating the unbiased CFR during the course of an
outbreak. (Nishiura et
al., 2009)
Figure 9
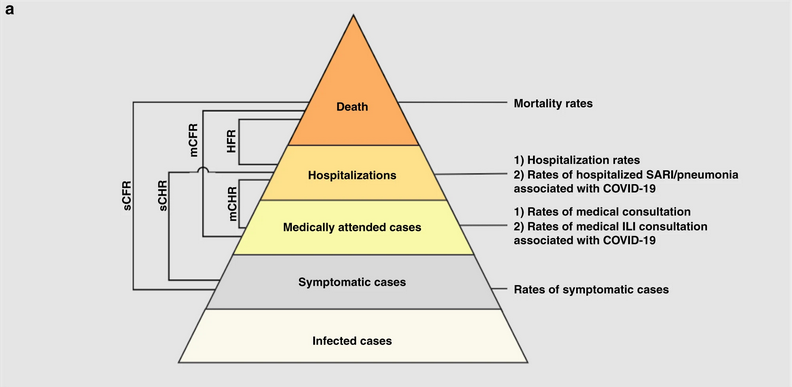
Severity levels of infections with SARS-CoV-2
and parameters of interest. Each level is assumed to be a subset of the
level below.
Figure 10
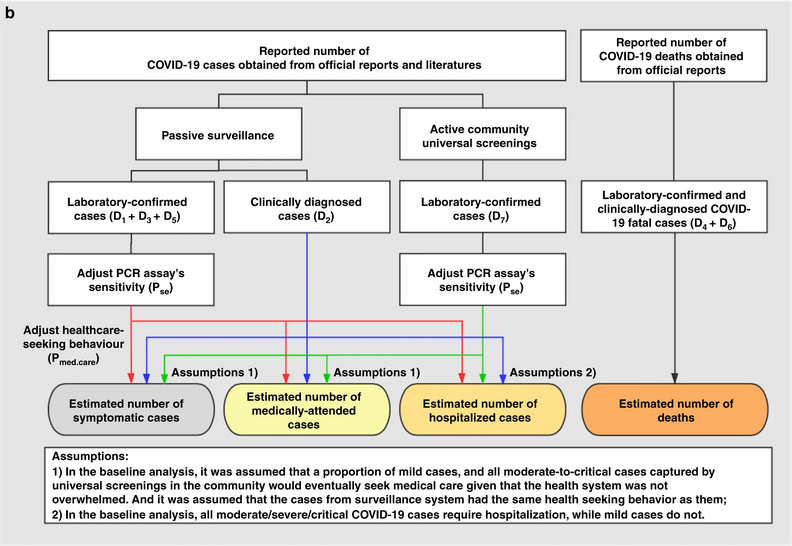
Schematic diagram of the baseline analyses. Red,
blue, and green arrows denote the data flow from laboratory-confirmed
cases of passive surveillance, clinically-diagnosed cases, and
laboratory-confirmed cases of active screenings.
Account for superspreading
Figure 1
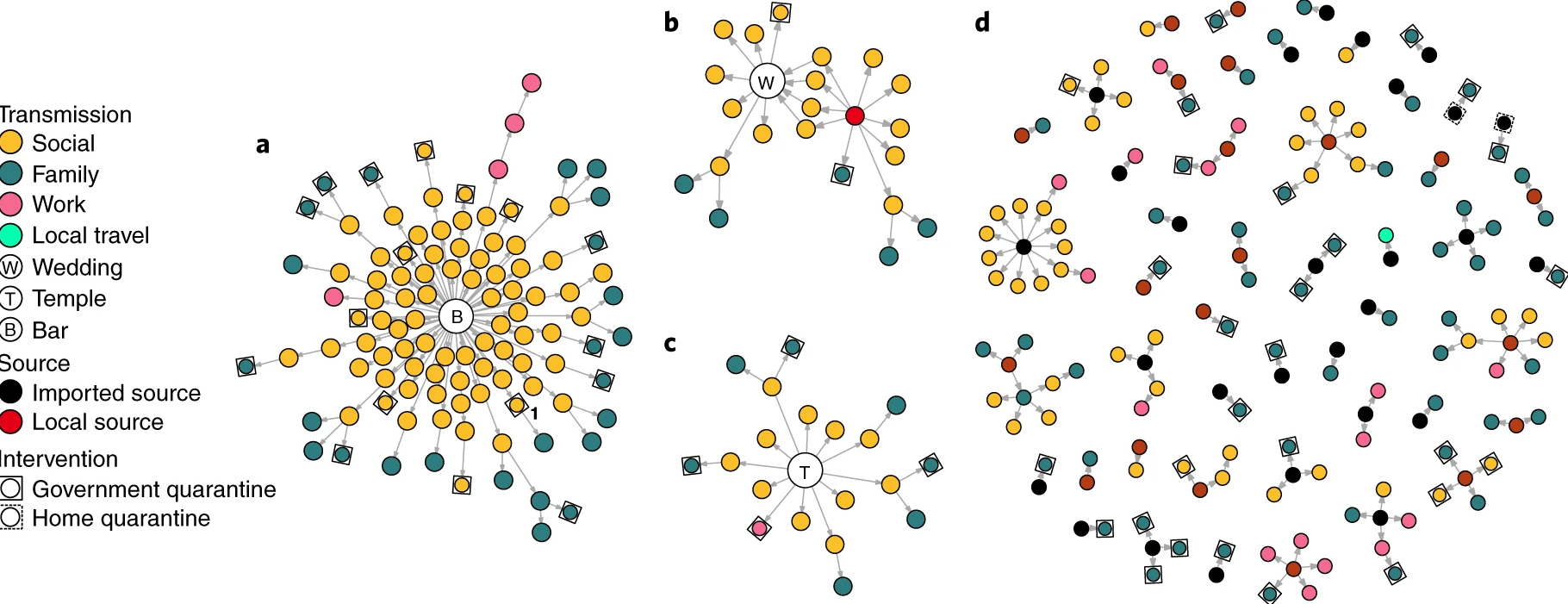
Chains of SARS-CoV-2 transmission in
Hong Kong initiated by local or imported cases.
(a), Transmission network of a cluster of cases traced
back to a collection of four bars across Hong Kong (n = 106).
(b), Transmission network associated with a wedding
without clear infector–infectee pairs but linked back to a preceding
social gathering and local source (n = 22). (c),
Transmission network associated with a temple cluster of undetermined
source (n = 19). (d), All other clusters of SARS-CoV-2
infections where the source and transmission chain could be determined
(Adam et
al., 2020).
Figure 2
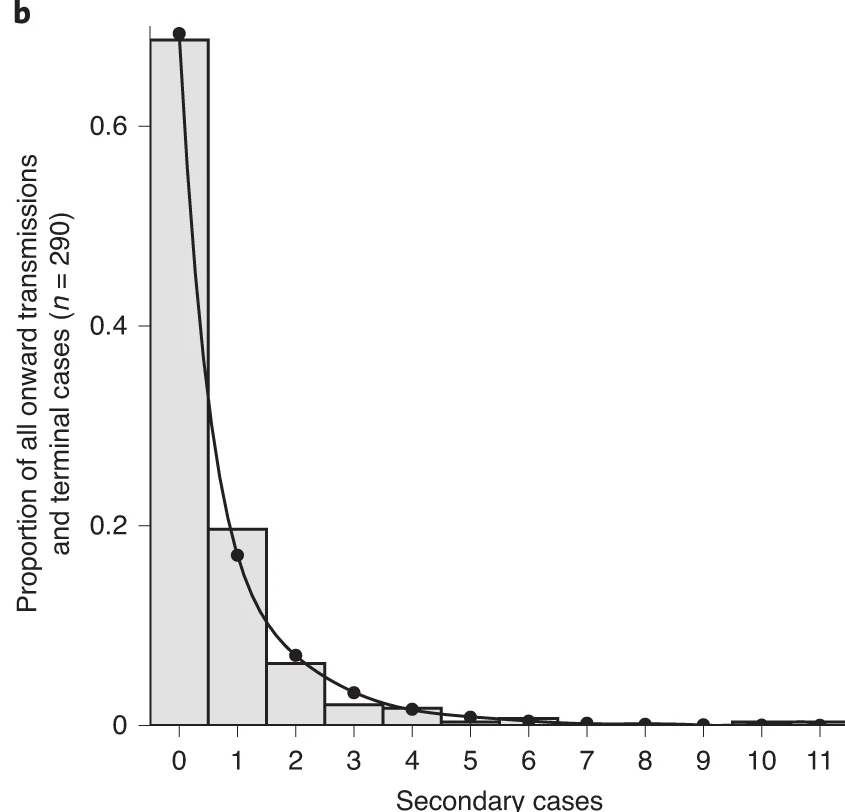
Observed offspring distribution of
SARS-CoV-2 transmission in Hong Kong. N = 91 SARS-CoV-2
infectors, N = 153 terminal infectees and N = 46 sporadic local cases.
Histogram bars indicate the proportion of onward transmission per amount
of secondary cases. Line corresponds to a fitted negative binomial
distribution (Adam et al.,
2020).
Figure 3
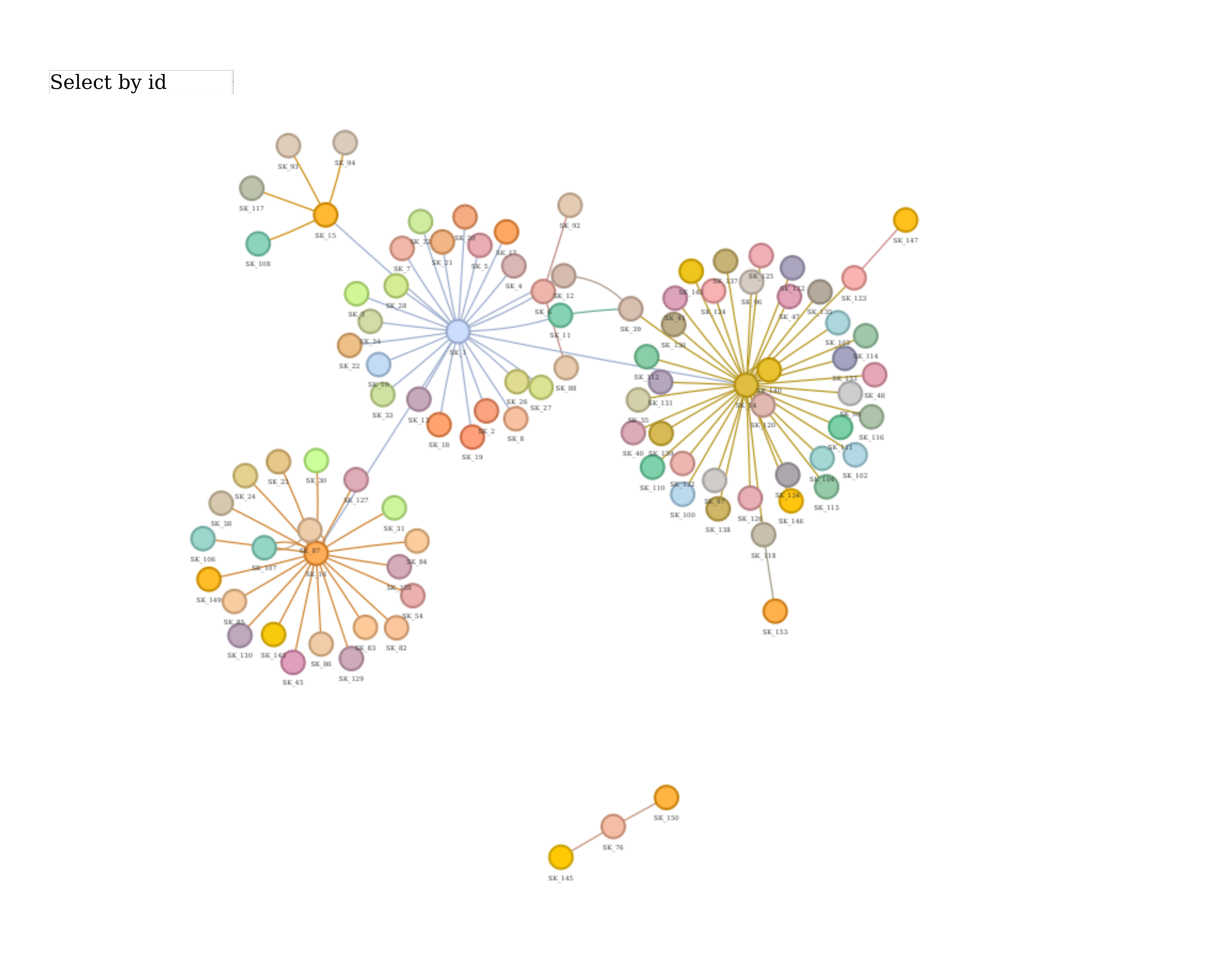
Figure 4
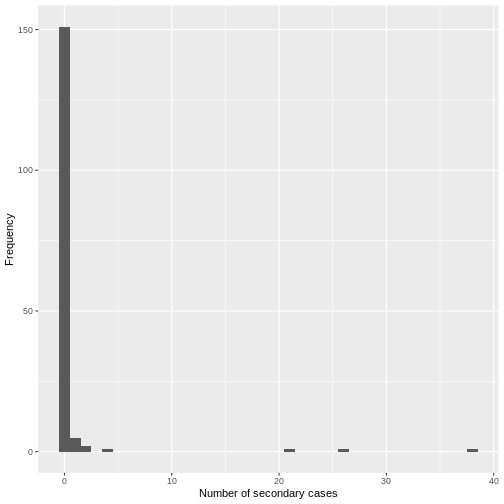
Figure 5
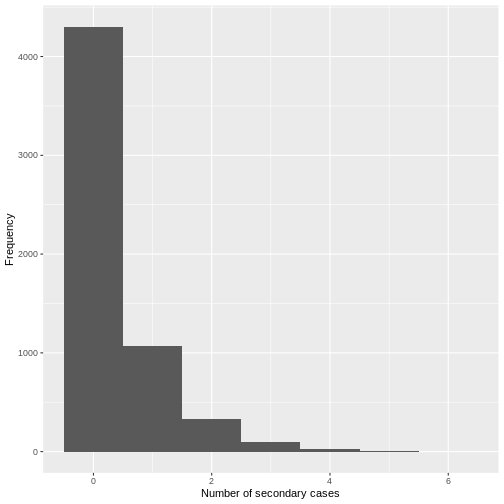
Figure 6
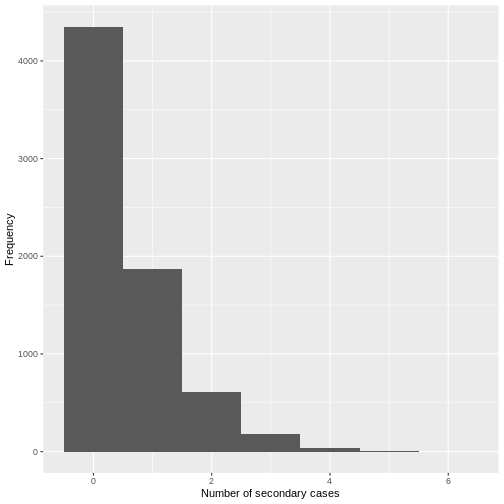
Figure 7
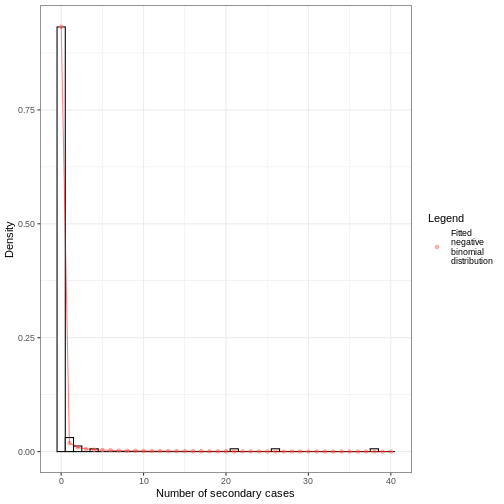
Figure 8
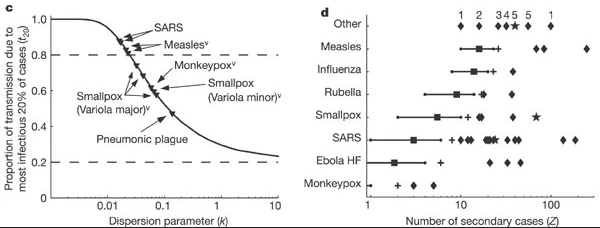
Evidence for variation in individual
reproductive number. (Left, c) Proportion of transmission
expected from the most infectious 20% of cases, for 10 outbreak or
surveillance data sets (triangles). Dashed lines show proportions
expected under the 20/80 rule (top) and homogeneity (bottom). (Right,
d), Reported superspreading events (SSEs; diamonds) relative to
estimated reproductive number R (squares) for twelve directly
transmitted infections. Crosses show the 99th-percentile proposed as
threshold for SSEs. (More figure details in Lloyd-Smith et al.,
2005)
Figure 9
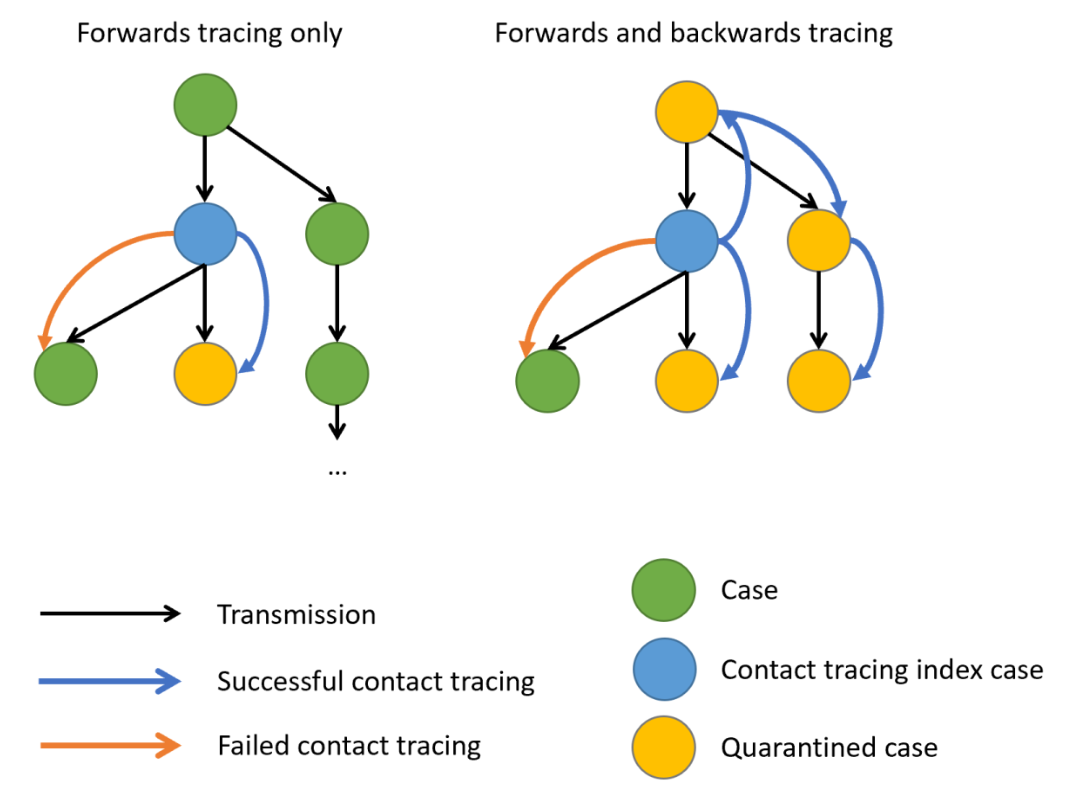
Schematic representation of contact tracing
strategies. Black arrows indicate the directions of transmission, blue
and Orange arrows, a successful or failed contact tracing,
respectivelly. When there is evidence of individual-level variation in
transmission, often resulting in superspreading, backward contact
tracing from the index case (blue circle) increase the probability to
find the primary case (green circle) or clusters with a larger fraction
of cases, potentially increasing the number of quarentined cases (yellow
circles). Claire
Blackmore, 2021
Figure 10
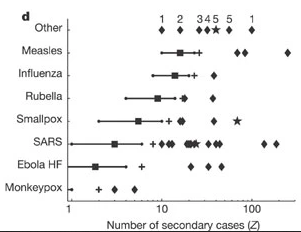
Reported superspreading events (SSEs; diamonds)
relative to estimated reproduction number R (squares) for twelve
directly transmitted infections. Lines show 5–95 percentile range of the
number of secondary cases following a Poisson distribution with lambda
equal to the reproduction number (\(Z∼Poisson(R)\)), and crosses show the
99th-percentile proposed as threshold for SSEs. Stars represent SSEs
caused by more than one source case. ‘Other’ diseases are: 1,
Streptococcus group A; 2, Lassa fever; 3, Mycoplasma pneumonia; 4,
pneumonic plague; 5, tuberculosis. R is not shown for ‘other’ diseases,
and is off-scale for monkeypox.
Simulate transmission chains
Figure 1
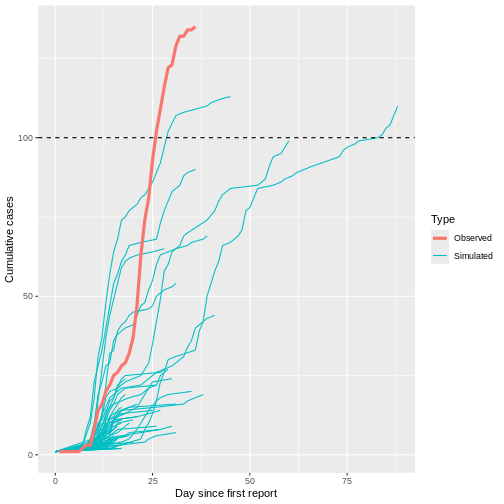
Figure 2
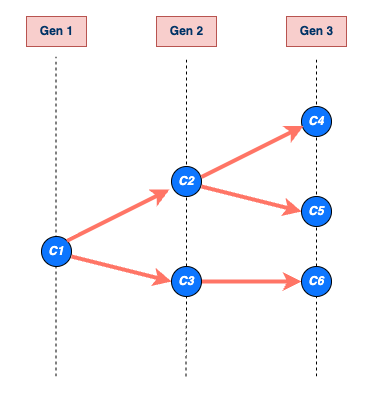
An example of a transmission chain
starting with a single case C1. Cases are represented by blue
circles and arrows indicating who infected whom. The chain grows through
generations Gen 1, Gen 2, and Gen 3, producing cases C2, C3, C4, C5, and
C6. The chain ends at generation Gen 3 with cases C4, C5, and C6. The
size of C1’s chain is 6, including C1 (that is, the sum of all blue
circles), and the length is 3, which includes Gen 1 (maximum number of
generations reached by C1’s chain) (Azam
& Funk, 2024).
Figure 3
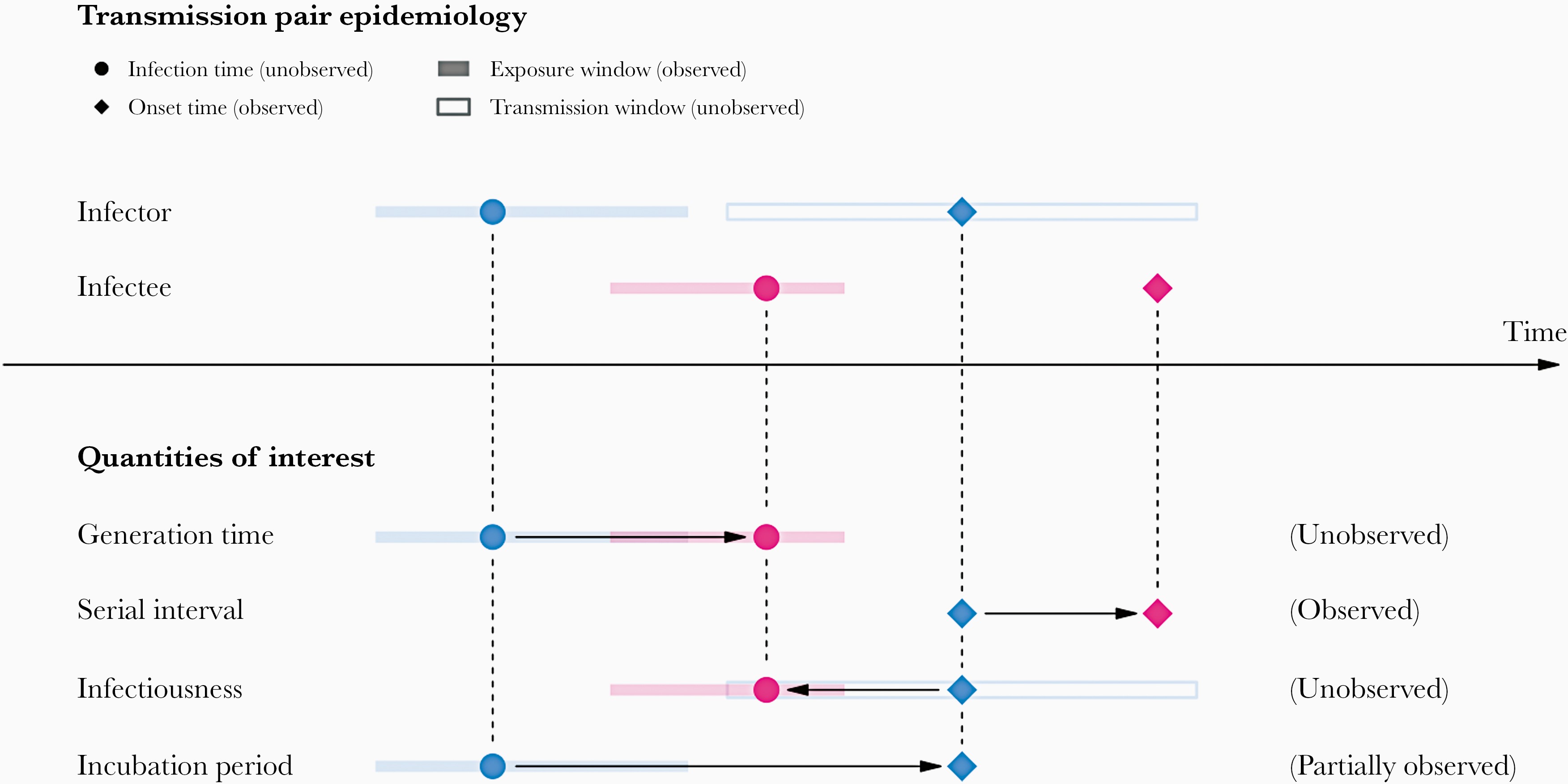
A schematic of the relationship of different
time periods of transmission between an infector and an infectee in a
transmission pair. Exposure window is defined as the time interval
having viral exposure, and transmission window is defined as the time
interval for onward transmission with respect to the infection time (Chung
Lau et al., 2021).
Figure 4
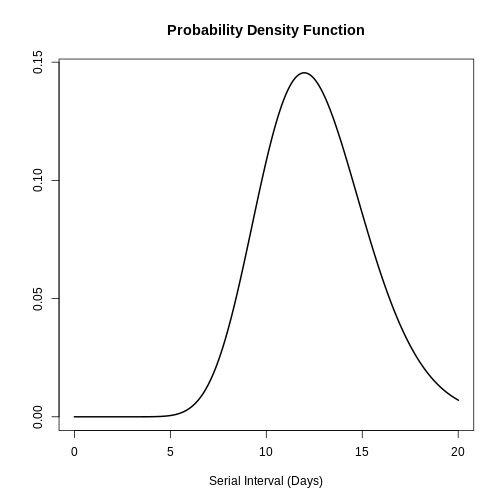
Figure 5
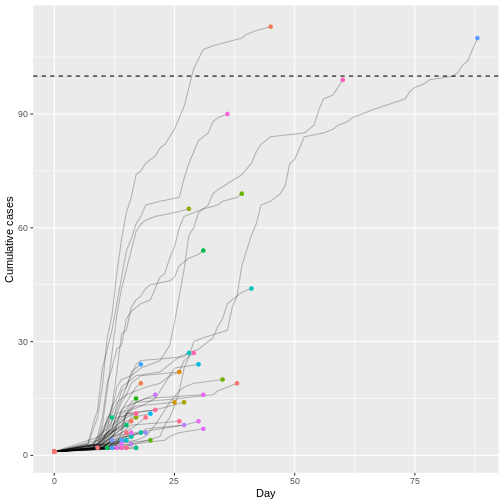
Figure 6
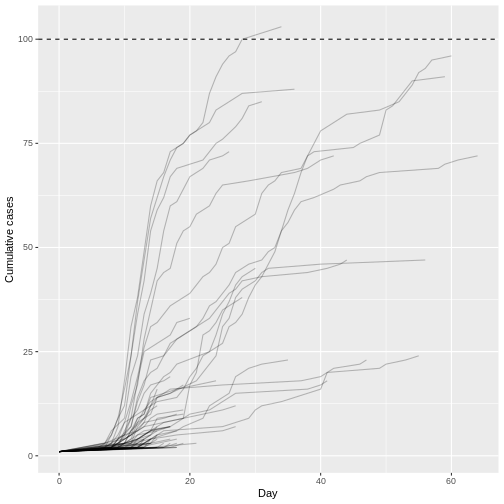
Figure 7
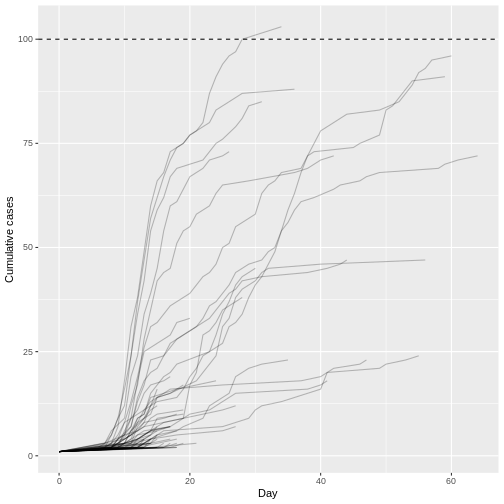
Figure 8
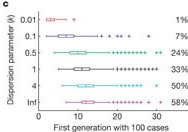
Growth of simulated outbreaks with R =
1.5 and one initial case, conditional on non-extinction. Boxes
show the median and interquartile range (IQR) of the first disease
generation with 100 cases; whiskers show the most extreme values within
1.5 × IQR of the boxes, and crosses show outliers. Percentages show the
proportion of 10,000 simulated outbreaks that reached the 100-case
threshold (Lloyd-Smith et al.,
2005).
Figure 9
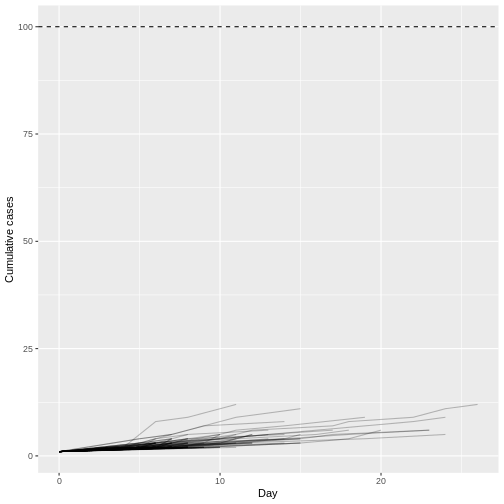
Figure 10
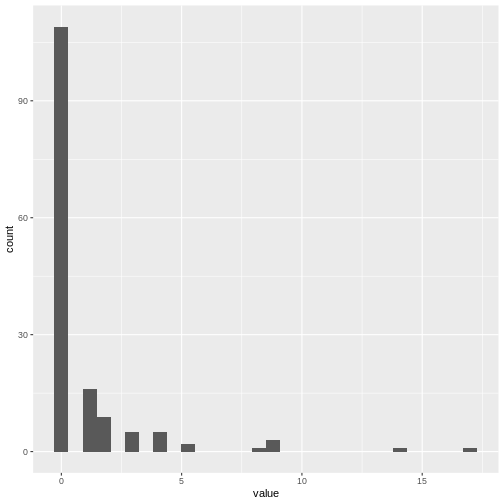
Figure 11
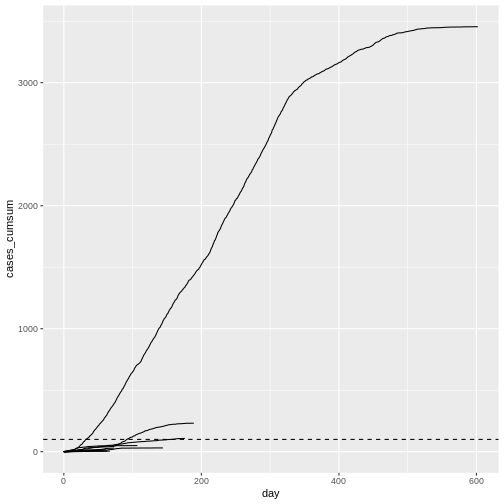